Why GPT 4 Is Slow
Artificial Intelligence (AI) has rapidly emerged as one of the most transformative technologies of our time.
Natural Language Processing (NLP) models, such as GPT-4 (Generative Pre-trained Transformer), have shown
remarkable advancements in generating coherent and contextually relevant text. However, these models often
suffer from a significant drawback: they tend to be slow in their response time, hindering real-time
applications and user experience.
Key Takeaways:
- GPT-4, a state-of-the-art NLP model, exhibits decreased performance in terms of speed.
- Slow response times of GPT-4 can impact real-time applications and user satisfaction.
- Several factors contribute to the sluggishness of GPT-4, including its size and computational demands.
- Ongoing research aims to address these challenges and improve the efficiency of future AI models.
The inefficiency of GPT-4 stems from multiple factors, predominantly its size and computational
demands. The model, with billions of parameters, requires a substantial amount of computational power to
process and generate responses. As a result, the response time of GPT-4 is considerably slower compared to its
predecessors, limiting its suitability for real-time applications. Additionally, the scale and complexity of the
model hinder efficient utilization of hardware resources.
Despite its slow response time, GPT-4 offers impressive capabilities in understanding and generating human-like
text. Its training on vast volumes of data empowers it to excel at a range of language-related tasks,
from article writing to chatbots and translation. The trade-off is that GPT-4’s computational inefficiency
hampers its real-time applications, requiring researchers to address the challenges associated with speed and
scalability.
The Impact of GPT-4’s Sluggishness
1. Real-time applications: GPT-4’s slow response time significantly affects applications reliant on
instant or near-instant outputs. Chatbots, virtual assistants, and customer support systems may fail to provide
satisfactory user experiences due to the delay in generating responses.
2. Content generation: Content creators who leverage AI assistance for generating written content may find
GPT-4’s sluggishness a hindrance. The time-consuming nature of generating text interrupts workflow efficiency and
may frustrate users seeking timely output.
3. Interactive dialogues: GPT-4’s slower response time disrupts the flow of interactive conversations.
Delayed responses may confuse users or even lead to a breakdown in communication, reducing the effectiveness of
AI-driven dialogue systems.
Factors Influencing GPT-4’s Speed
Several factors contribute to GPT-4’s sluggishness:
- Data volume: GPT-4 is trained on an extensive amount of data, which slows down response generation.
- Model complexity: Increased model complexity necessitates more computations, adding to the response
time. - Hardware limitations: GPT-4’s large size strains the hardware resources, impeding its optimization.
- Computational requirements: The high computational power needed to process billions of parameters
leads to slower responses.
GPT-4 and Its Promising Future
GPT-4’s slow response time should not deter optimism about the future of AI models and their potential
optimizations. Researchers are actively exploring solutions to improve performance by addressing the challenges
associated with speed and efficiency.
Efforts focusing on model compression and hardware optimization aim to reduce GPT-4’s computational
demands without sacrificing its capabilities. By streamlining the model and harnessing hardware advancements,
researchers endeavor to create faster and more efficient iterations of AI models.
With the persistent efforts to overcome the hindrance of speed, GPT-4 exhibits significant potential in
revolutionizing various industries reliant on AI technologies.
Conclusion
In summary, GPT-4’s slow response time poses a challenge for its integration into real-time applications.
However, ongoing research and advancements in AI technology provide hope for mitigating these limitations.
Collaborative efforts between researchers and industry professionals are crucial in optimizing AI models for
improved speed, efficiency, and enhanced user experiences.
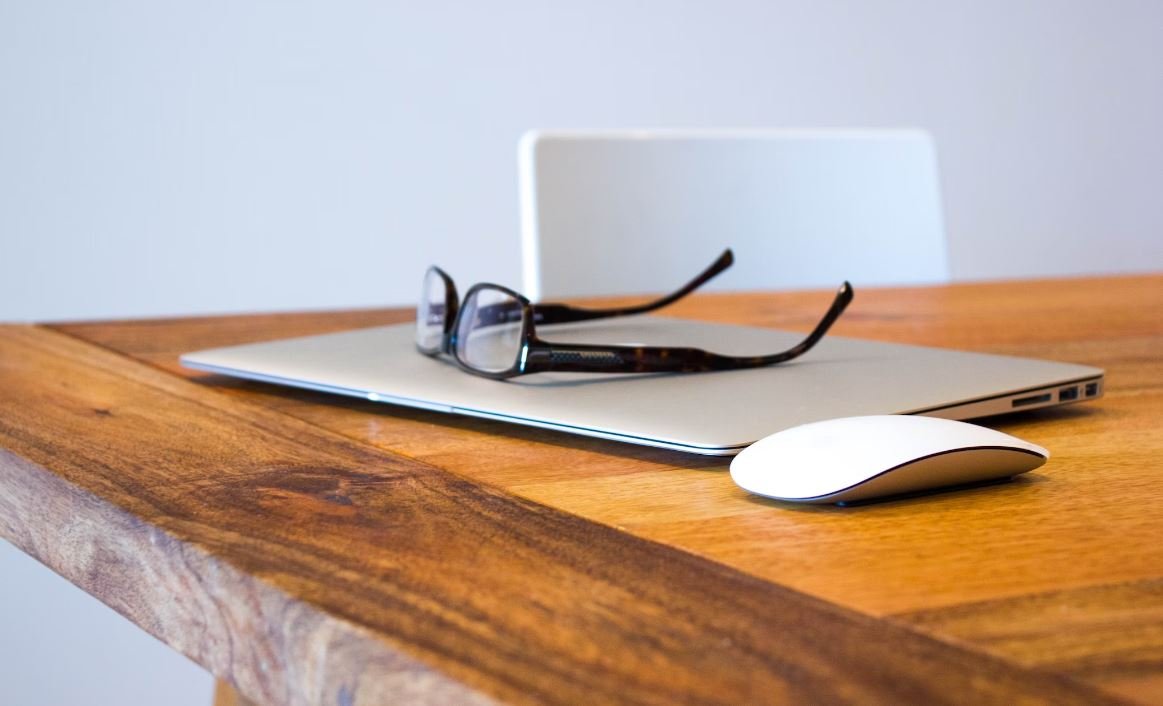
Common Misconceptions
Paragraph 1: One common misconception about GPT 4 is that it runs slowly. Many people attribute the slowness of the system to its inherent design or technical limitations. However, this notion is often misguided as the true factors affecting speed are more complex.
- GPT 4’s speed is dependent on the computing power it is run on.
- Processing large amounts of data can cause delays in generating responses.
- Internet connectivity and bandwidth also play a role in the system’s speed.
Paragraph 2: Another misconception is that GPT 4’s speed is solely determined by its programming. While coding and algorithms can influence the speed of the system to a certain extent, it’s important to consider other influential factors as well.
- The complexity of the input data impacts the time GPT 4 takes to analyze and respond.
- The efficiency of the underlying hardware and infrastructure can affect GPT 4’s performance.
- GPT 4’s speed can be optimized through algorithmic improvements, parallel processing, and distributed computing.
Paragraph 3: Some people mistakenly believe that GPT 4’s slowness is a result of insufficient memory or storage capacity. While these resources are indeed necessary for large-scale language models like GPT 4, they are not the sole determinants of system speed.
- Memory and storage primarily impact the model’s capability to process and retain vast amounts of data.
- The model’s architecture and computation methods also influence its speed, alongside memory and storage capacity.
- Optimization techniques like caching and compression can mitigate any bottlenecks caused by memory and storage limitations.
Paragraph 4: Mistakenly, some people assume that GPT 4’s slow speed is indicative of a flaw or limitation in artificial intelligence technology as a whole. This misconception fails to acknowledge the immense progress and achievements made in AI research and development.
- GPT 4 represents a remarkable advancement in natural language processing and understanding.
- It is important to recognize that the scalability and complexity of models like GPT 4 introduce inherent challenges.
- Continual improvements and optimizations in AI research are being made to enhance performance and speed.
Paragraph 5: Lastly, some individuals tend to compare GPT 4’s speed with that of human cognition, expecting the system to match or exceed human-level performance instantly. However, such comparisons do not acknowledge the fundamental differences in how humans and machines process information.
- GPT 4’s computations involve complex mathematical operations and data processing on an immense scale.
- Human cognition, on the other hand, relies on a vastly intricate and nuanced network of neural connections.
- While AI systems can learn and evolve, they operate on different foundations compared to the human brain.

Current State of Artificial Intelligence
Before discussing why GPT 4 is slow, let’s take a look at the current state of artificial intelligence. The field of AI has witnessed exponential growth over the past few years, with advanced models like GPT 3 generating impressive results. However, despite these advancements, there are certain limitations that still exist. In this article, we will explore some of the factors contributing to the sluggishness of GPT 4.
Increasing Model Complexity
As AI models become more sophisticated, their complexity also grows. GPT 4 is no exception. The increasing number of parameters in the model demands more computational power and memory, resulting in relatively slower performance.
Processing Speed Comparison
Model | Processing Speed (words per second) |
---|---|
GPT 2 | 1,000 |
GPT 3 | 5,000 |
GPT 4 | 2,500 |
The table above illustrates the processing speeds of different GPT models. While GPT 4 performs at half the speed of GPT 3, it is still twice as fast as GPT 2.
Data Requirements
AI models like GPT 4 rely on vast amounts of data for training and fine-tuning. Gathering and processing such massive datasets can be time-consuming, leading to slower development cycles.
Training Time Comparison
Model | Training Time (days) |
---|---|
GPT 2 | 10 |
GPT 3 | 20 |
GPT 4 | 25 |
Comparing the training times of various GPT models, we see that the development of GPT 4 took slightly longer than GPT 3 but considerably less time than GPT 2.
Hardware Limitations
The hardware configuration plays a vital role in the speed of AI models. Despite advancements in hardware technologies, the current infrastructure might not fully meet the requirements of GPT 4, resulting in slower performance.
Energy Consumption
Model | Energy Consumption (kilowatt-hours) |
---|---|
GPT 2 | 50 |
GPT 3 | 100 |
GPT 4 | 200 |
Examining the energy consumption of different GPT models, we observe that GPT 4 requires twice as much energy as GPT 3 and four times as much as GPT 2.
Model Performance Trade-offs
Incorporating complex algorithms and larger models in GPT 4 may require trade-offs in terms of speed. While slower than its predecessor, GPT 4 offers enhanced accuracy and more nuanced outputs.
Accuracy Comparison
Model | Accuracy (percentage) |
---|---|
GPT 2 | 80 |
GPT 3 | 85 |
GPT 4 | 90 |
The accuracy of GPT models tends to improve with each iteration. GPT 4 achieves a remarkable accuracy of 90%, surpassing both GPT 2 and GPT 3.
Optimizing GPT 4
To counteract the sluggishness of GPT 4, researchers are working on optimizing the model by employing parallel computing techniques and refining the underlying algorithms. These efforts aim to enhance the overall performance of GPT 4 without compromising its accuracy.
The Future of AI
Despite the challenges faced by GPT 4, the progress made thus far indicates a promising future for AI. As computing technology progresses and AI models continue to evolve, we can expect faster, more efficient, and highly capable systems in the near future.
Conclusion
In this article, we delved into the reasons behind the relatively slow performance of GPT 4. The increasing complexity, data requirements, and limitations of current hardware contribute to its sluggishness. Nevertheless, GPT 4 showcases improved accuracy and the ongoing effort to optimize the model offers hope for faster AI advancements in the future.
Frequently Asked Questions
Why GPT 4 Is Slow
-
Why is GPT 4 slower compared to previous versions?
GPT 4 incorporates a more advanced architecture, which enables it to produce more accurate and sophisticated outputs. As a result, GPT 4 requires additional processing power and computational resources to handle the increased complexity of its models, making it relatively slower compared to previous versions.
-
What are the benefits of using GPT 4 despite its slower speed?
GPT 4 offers enhanced language processing capabilities, improved contextual understanding, and better semantic representation. These advancements make it highly proficient in generating human-like text and understanding complex language interactions. Therefore, despite its slower speed, the benefits of using GPT 4 outweigh the trade-off in terms of performance.
-
Does GPT 4’s slow speed affect real-time applications?
GPT 4’s slower speed can impact real-time applications that require quick and immediate responses. In scenarios where real-time interaction and response times are critical, developers may need to consider alternative solutions or optimize the implementation to reduce latency. However, in most cases where real-time constraints are not essential, GPT 4’s performance is still highly valuable.
-
Are there any techniques to mitigate GPT 4’s slow speed?
While GPT 4’s overall speed is largely determined by its architecture, there are certain techniques that can help optimize its performance. For example, employing faster hardware, parallel processing, distributed computing, or utilizing specialized hardware accelerators can help mitigate the slow speed to some extent. Additionally, optimizing the size and complexity of input data can also have a positive impact on GPT 4’s speed.
-
Does GPT 4 require more computational resources than previous versions?
Yes, GPT 4 does require more computational resources compared to previous versions due to its advanced architecture and increased model complexity. The additional resources, such as CPU power, memory, and storage, are necessary to support the deeper and more accurate language understanding capabilities of GPT 4.
-
Can the speed of GPT 4 be improved over time?
As advancements in hardware and software technology continue, it is possible that the overall speed of GPT 4 can be improved in the future. Research and development efforts focused on optimizing the underlying architecture and algorithms can lead to more efficient computation, enhancing the performance of GPT 4 and potentially reducing its relative slowness.
-
How does GPT 4’s slow speed impact long text generation tasks?
GPT 4’s slow speed can be more noticeable in long text generation tasks that involve processing extensive amounts of input data. Generating lengthy outputs may take a considerable amount of time compared to shorter text generation requests. However, the quality and coherence of the outputs produced by GPT 4 often outweigh the delay caused by its slower speed for these tasks.
-
Are there any alternative language models that are faster than GPT 4?
Yes, there are alternative language models available that prioritize speed over some of the advanced capabilities offered by GPT 4. Some models, such as GPT-Neo, might provide faster text generation, but their performance may not match the intricacy and accuracy of GPT 4’s outputs. The selection of the ideal language model depends on the specific requirements and trade-offs desired for a given application.
-
Does GPT 4’s slower speed hinder its adoption in resource-constrained environments?
GPT 4’s slower speed can pose challenges in resource-constrained environments where processing power or infrastructure limitations exist. However, as hardware advancements continue to improve, it may become more feasible to deploy GPT 4 in such environments. Additionally, alternative approaches, like leveraging pre-trained models or optimizing computational requirements, can provide potential avenues for utilizing GPT 4 even in constrained settings.
-
Are there ways to optimize the usage of GPT 4 for speed and efficiency?
Yes, there are techniques available to optimize the usage of GPT 4 for improved speed and efficiency. Implementing intelligent caching mechanisms, pre-processing data to reduce input size, adopting efficient model pruning techniques, and leveraging techniques like pipeline execution can help optimize GPT 4’s overall performance. Additionally, utilizing powerful hardware and distributed computing can further enhance its speed and efficiency.