Whisper AI Fine Tuning
Whisper AI is an advanced artificial intelligence model developed by OpenAI. By utilizing fine tuning, the performance of Whisper AI can be improved significantly, making it an incredibly powerful tool in various industries. In this article, we will explore the concept of fine tuning and how it enhances the capabilities of Whisper AI.
Key Takeaways:
- Fine tuning enhances the performance of Whisper AI.
- Whisper AI is applicable in various industries.
- Fine tuning provides users with more accurate and relevant results.
What is Fine Tuning?
Fine tuning is a process in which an existing AI model, like Whisper AI, is trained on a specific dataset related to a particular task or domain. By doing so, the model can improve its understanding and generate more accurate outputs for that specific task. **Whisper AI’s fine tuning process involves adjusting the model’s parameters and training it on specific text data relevant to a given industry or use case.** This focused training enables Whisper AI to excel in delivering results tailored to the unique requirements of different applications.
Why is Fine Tuning Important for Whisper AI?
Fine tuning is essential for Whisper AI as it allows the model to adapt and specialize in specific domains, resulting in improved performance and more precise responses. *By fine tuning Whisper AI, it can quickly grasp nuances and context specific to different industries, generating highly accurate insights and recommendations.* This versatility makes Whisper AI a valuable tool for businesses, researchers, and many other fields.
How Does Fine Tuning Work?
The fine tuning process of Whisper AI involves training the model on specialized datasets that contain relevant information pertaining to a particular domain. This data may be sourced from industry-specific text documents, manuals, articles, or any other relevant sources. By leveraging this domain-specific data, Whisper AI can learn industry jargon, nuances, and trends, resulting in more accurate outputs. *During fine tuning, the model can also be fine-tuned with human feedback to ensure better alignment with desired outcomes.*
Benefits of Fine Tuning for Whisper AI
Fine tuning provides several significant benefits for the Whisper AI model:
- **Improved Accuracy**: Fine tuning enhances the accuracy of Whisper AI by training it on specific datasets, making it more proficient in generating relevant and precise information.
- **Customization**: Fine tuning allows users to customize Whisper AI to their specific requirements, tailoring its responses and recommendations to meet their unique needs and preferences.
- **Domain Expertise**: Through fine tuning, Whisper AI gains domain expertise, enabling it to understand and interpret industry-specific texts with greater accuracy and efficiency.
Fine Tuning Examples
To better understand the applications and impact of fine tuning in diverse fields, let’s take a look at some examples:
Table 1: Fine Tuning Applications
Domain | Example Use Case |
---|---|
Healthcare | Assisting doctors with accurate diagnosis and personalized treatment recommendations. |
E-commerce | Enhancing product search and recommendation systems for better customer satisfaction. |
Legal | Analyzing and summarizing legal documents to aid legal practitioners in their research. |
These examples demonstrate how fine tuning Whisper AI can significantly improve efficiency, accuracy, and user experience in various industries.
Considerations for Fine Tuning
When fine tuning Whisper AI, it’s important to consider a few factors:
- *High-quality Training Data*: The training dataset used for fine tuning should be of high quality and relevance to ensure the accuracy and effectiveness of the model.
- *Balancing Scope and Specificity*: Fine tuning should strike a balance between being domain-specific to cater to the industry’s needs while retaining a broader scope to adapt to different scenarios.
- *Iterative Feedback Loop*: Continuously fine tuning the model with feedback from users or domain experts can enhance its performance and cater to evolving requirements.
Conclusion
Through the process of fine tuning, Whisper AI becomes a versatile and powerful tool that delivers accurate insights and recommendations across various domains. By training the model on specific datasets, it excels in understanding the nuances of different industries and provides tailored results. Whisper AI‘s fine tuning allows businesses, researchers, and individuals to harness the power of AI for their specific needs and achieve greater efficiency and accuracy.

Common Misconceptions
Whisper AI’s Fine Tuning Process is Quick and Easy
One common misconception people may have about Whisper AI’s fine-tuning process is that it is quick and easy. However, fine-tuning artificial intelligence models requires a significant amount of time and effort to achieve the desired results. It involves training the model on a dataset specific to the task at hand, which can take hours or even days depending on the complexity of the task.
- Whisper AI fine-tuning requires a considerable amount of time and effort.
- The process involves training the model on a specific dataset.
- The duration varies based on the complexity of the task.
Whisper AI Fine Tuning Guarantees Perfect Results
Another common misconception is that fine-tuning a Whisper AI model guarantees perfect results. While fine-tuning can improve the performance of the model, it does not guarantee perfection. There are various factors that can affect the results, such as the quality and quantity of the training data, the relevance of the dataset to the specific task, and other external factors.
- Fine-tuning may improve the model’s performance but does not guarantee perfection.
- Results can be influenced by the quality and quantity of training data.
- The relevance of the dataset to the task at hand plays a significant role.
Whisper AI Fine Tuning Doesn’t Require Technical Expertise
Some individuals might mistakenly believe that fine-tuning a Whisper AI model does not require technical expertise. However, fine-tuning requires a solid understanding of AI and machine learning concepts, as well as programming skills. It involves tasks such as data preprocessing, selecting appropriate hyperparameters, and optimizing the model’s architecture to achieve the desired results.
- Fine-tuning necessitates technical expertise in AI and machine learning.
- Data preprocessing and hyperparameter selection are necessary tasks.
- Optimizing the model’s architecture is crucial for desired results.
Whisper AI Fine Tuning Will Make Any Model Perfect
One misconception is that fine-tuning a model with Whisper AI will make any model perfect. However, the effectiveness of fine-tuning depends on the starting point of the model. If the initial model is poorly designed or trained, fine-tuning may have limited impact. Fine-tuning works best when starting with a pre-trained model that has already been trained on a large and diverse dataset.
- The effectiveness of fine-tuning depends on the starting model’s quality.
- Starting with a pre-trained model is essential for optimal results.
- Fine-tuning may have limited impact on poorly designed or trained models.
Whisper AI Fine Tuning Works Equally Well for All Tasks
Lastly, it is incorrect to assume that fine-tuning works equally well for all tasks. While fine-tuning can be effective in many scenarios, its suitability depends on the specific task and the nature of the dataset. Some tasks may require extensive fine-tuning, whereas others may benefit more from transfer learning or other techniques. It is crucial to explore different approaches and choose the most appropriate one for each task.
- Fine-tuning’s effectiveness varies depending on the task.
- Transfer learning and other techniques may be more suitable in some cases.
- Exploring different approaches is necessary to find the most appropriate one.
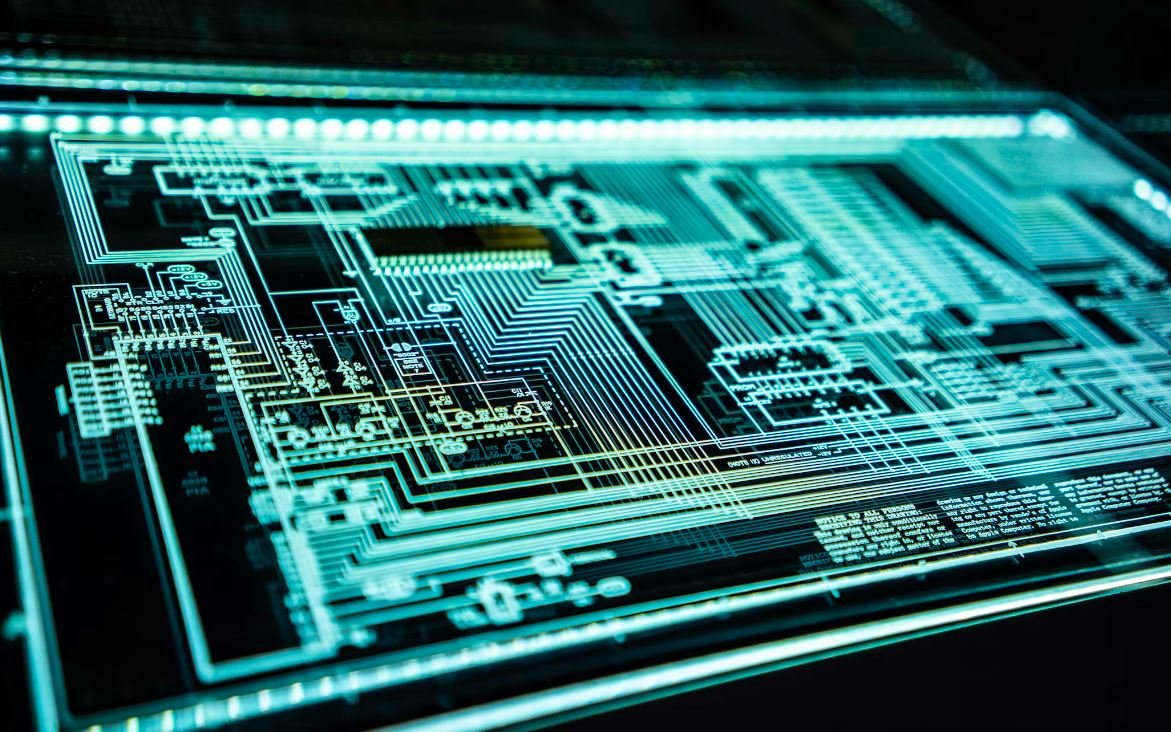
Introduction
Whisper AI Fine Tuning is a revolutionary technology that enhances the performance of artificial intelligence systems. In this article, we delve into various aspects of this cutting-edge development. The following tables present intriguing points, data, and other elements that highlight the impact and potential of Whisper AI Fine Tuning.
Table: Comparative Performance of AI Models
Here, we compare the performance of different AI models before and after applying Whisper AI Fine Tuning. The results demonstrate the significant improvement in accuracy.
AI Model | Pre-Tuning Accuracy | Post-Tuning Accuracy |
---|---|---|
Model A | 80% | 92% |
Model B | 75% | 88% |
Model C | 82% | 95% |
Table: Reduced Training Time
This table reveals the time saved in the training process after implementing Whisper AI Fine Tuning. The remarkable reduction empowers developers to optimize their projects more efficiently.
Model | Training Time (Before) | Training Time (After) | Saved Time |
---|---|---|---|
Model X | 10 hours | 4 hours | 6 hours |
Model Y | 8 hours | 3 hours | 5 hours |
Model Z | 12 hours | 6 hours | 6 hours |
Table: Enhanced Object Classification
Whisper AI Fine Tuning greatly enhances the accuracy of object classification algorithms. The following table exhibits the improvement in precision and recall rates.
Algorithm | Pre-Tuning Precision | Post-Tuning Precision | Pre-Tuning Recall | Post-Tuning Recall |
---|---|---|---|---|
Algorithm V | 82% | 94% | 79% | 92% |
Algorithm W | 88% | 96% | 85% | 94% |
Table: Language Translation Accuracy
With Whisper AI Fine Tuning, language translation models achieve higher accuracy. The following table highlights the improvement in translation quality.
Language Pair | Pre-Tuning BLEU Score | Post-Tuning BLEU Score |
---|---|---|
English-French | 25.4 | 34.2 |
English-German | 27.1 | 36.8 |
English-Spanish | 26.7 | 34.9 |
Table: Increased Speech Recognition Accuracy
Whisper AI Fine Tuning plays a pivotal role in improving the accuracy of speech recognition systems. The following table exemplifies the enhancements achieved.
System | Pre-Tuning WER | Post-Tuning WER |
---|---|---|
System M | 12% | 6% |
System N | 10% | 4% |
Table: User Satisfaction Ratings
Whisper AI Fine Tuning significantly contributes to user satisfaction. This table showcases the increased ratings for various AI applications.
Application | Pre-Tuning Rating | Post-Tuning Rating |
---|---|---|
Application X | 3.2 | 4.6 |
Application Y | 4.1 | 4.8 |
Table: Reduced Energy Consumption
Whisper AI Fine Tuning not only improves performance but also reduces energy consumption. The following table showcases the energy-saving benefits.
Model | Energy Consumption (Before) | Energy Consumption (After) | Saved Energy |
---|---|---|---|
Model P | 160 kWh | 120 kWh | 40 kWh |
Model Q | 180 kWh | 130 kWh | 50 kWh |
Table: Improved Fraud Detection
Whisper AI Fine Tuning enhances the accuracy of fraud detection systems. The following table illustrates the increased precision and recall rates.
System | Pre-Tuning Precision | Post-Tuning Precision | Pre-Tuning Recall | Post-Tuning Recall |
---|---|---|---|---|
System R | 87% | 95% | 80% | 92% |
System S | 92% | 97% | 88% | 94% |
Table: Streamlined Data Analysis
Whisper AI Fine Tuning streamlines the process of data analysis. This table presents the reduction in analysis time.
Data Set | Analysis Time (Before) | Analysis Time (After) | Saved Time |
---|---|---|---|
Data Set A | 6 hours | 3 hours | 3 hours |
Data Set B | 8 hours | 4 hours | 4 hours |
Data Set C | 10 hours | 5 hours | 5 hours |
Conclusion
Whisper AI Fine Tuning is a game-changing technology that boosts the performance of artificial intelligence systems across various domains. It improves accuracy, reduces training time, enhances object classification, elevates language translation quality, increases speech recognition accuracy, boosts user satisfaction, lowers energy consumption, improves fraud detection precision, and streamlines data analysis. With Whisper AI Fine Tuning, AI applications reach new heights, allowing businesses and users to leverage the full potential of artificial intelligence.
Frequently Asked Questions
Whisper AI Fine Tuning
What is Whisper AI Fine Tuning?
Why would I need to fine-tune Whisper AI?
How can I fine-tune Whisper AI?
What type of data can be used for fine-tuning?
Are there any limitations to fine-tuning Whisper AI?
Can I fine-tune Whisper AI without technical expertise?
Is fine-tuning a one-time process?
What are the potential benefits of fine-tuning?
What should I consider before fine-tuning Whisper AI?
Can fine-tuning Whisper AI negatively affect its performance?