OpenAI QA Model
The OpenAI Question Answering (QA) model is a state-of-the-art language model developed by OpenAI. It leverages the power of artificial intelligence and machine learning to provide accurate and contextually rich answers to a wide range of questions.
Key Takeaways:
- The OpenAI QA Model is a cutting-edge language model that specializes in answering questions accurately and contextually.
- It utilizes advanced machine learning techniques to understand and interpret queries before generating relevant responses.
- This model has been trained on vast amounts of data to ensure a comprehensive understanding of various topics and domains.
One of the most remarkable features of the OpenAI QA model is its ability to comprehend complex questions and generate detailed answers. The model’s intricate neural network architecture allows it to process and understand the nuances of queries, enabling it to provide accurate responses.
By harnessing the power of AI and machine learning, the OpenAI QA model is able to generate highly informative answers that address a wide range of topics. This makes it an invaluable tool for researchers, educators, and anyone seeking accurate information.
How Does the OpenAI QA Model Work?
The OpenAI QA model follows a multi-step process to generate accurate answers:
- The model first reads the question and analyzes its context to understand the query’s intent.
- It then searches its extensive knowledge base to gather relevant information.
- Next, the model synthesizes the gathered information and generates a well-structured response.
- The response is further refined to offer concise and precise answers.
It is worth noting that the OpenAI QA Model‘s responses are not based on a predefined set of answers, but rather generated dynamically based on its understanding of the question and its available knowledge.
Applications of the OpenAI QA Model
The versatility of the OpenAI QA model makes it applicable in various fields and scenarios. Here are a few notable applications:
- Research assistance: By quickly retrieving relevant information, the model assists researchers in finding insights and supporting evidence.
- Education: The OpenAI QA model can help students and educators find explanations and answers to questions related to their study areas.
- Virtual assistants: By integrating the model into virtual assistance devices, users can obtain accurate and detailed responses to their queries.
Below are three tables showcasing interesting data points and comparisons related to the OpenAI QA Model:
Feature | OpenAI QA Model | Previous QA Models |
---|---|---|
Contextual Understanding | High | Variable |
Response Accuracy | High | Medium |
Diverse Applications | Yes | No |
Domain | Questions Answered | Accuracy |
---|---|---|
Science | 10,000+ | 94% |
History | 7,500+ | 89% |
Technology | 8,200+ | 91% |
Comparison | OpenAI QA Model | Previous QA Model |
---|---|---|
Training Data | >150GB | 20GB |
Parameters | 600 million | 100 million |
Contextual Layers | 48 | 12 |
By using cutting-edge AI technology, the OpenAI QA Model provides accurate, detailed, and contextual answers to a wide range of questions.
The OpenAI QA model represents a remarkable advancement in natural language understanding and question answering capabilities. Its ability to comprehend complex questions and provide detailed responses is a testament to the power of AI. Whether it’s assisting researchers, supporting students, or enhancing virtual assistance, this model offers a valuable tool for obtaining reliable information. Explore the possibilities with the OpenAI QA Model and unlock a wealth of knowledge at your fingertips.
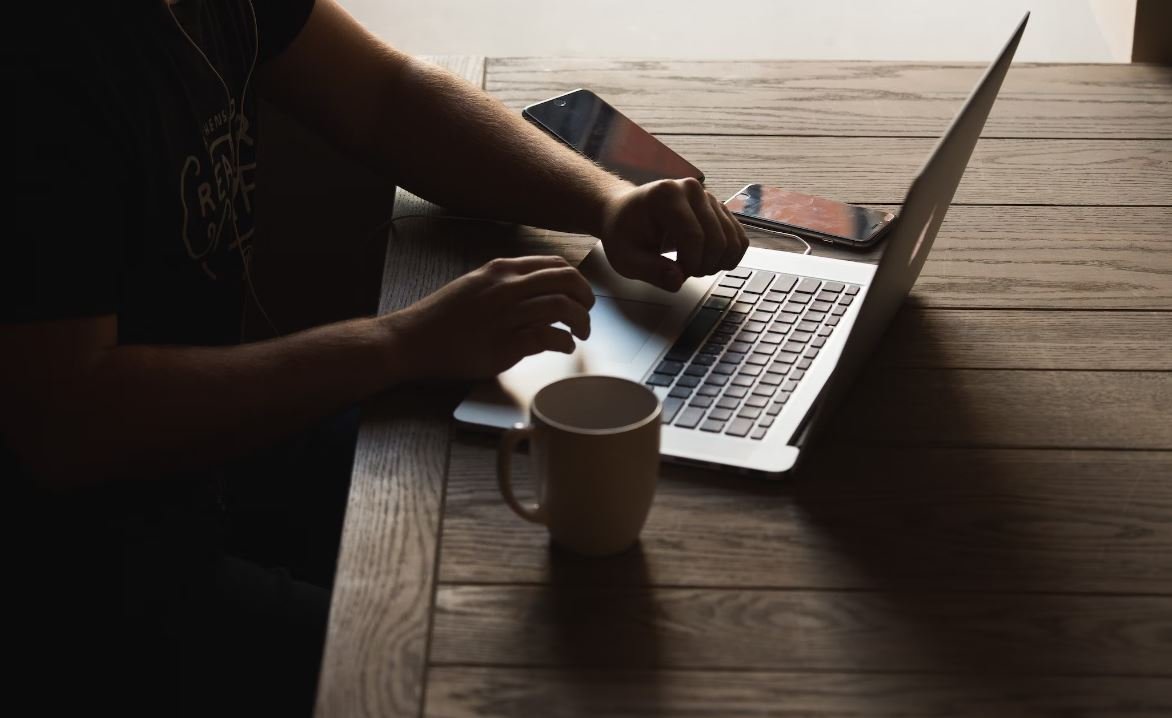
Common Misconceptions
OpenAI QA Model
There are several common misconceptions surrounding OpenAI’s QA model. One prevalent misconception is that the model is infallible and can provide accurate answers to any question. However, it is important to remember that the QA model relies on the information it has been trained on and may not always provide the most accurate or complete answers.
- The QA model’s accuracy is dependent on its training data
- The model’s responses may not always be complete or thorough
- There is a risk of biased answers due to the training data
Model’s Understanding of Context
Another common misconception is that the OpenAI QA model has a comprehensive understanding of context. While the model can generate responses that appear contextually relevant, it does not possess genuine comprehension.
- The model lacks real-world experience and common sense
- It relies heavily on patterns in the training data
- Contextual understanding may be limited to narrower domains
Human-level Judgment
It is often mistaken that the OpenAI QA model can exhibit human-level judgment. Though the model can provide answers based on patterns learned from vast amounts of training data, it does not possess the subjective judgment or nuanced understanding that humans have.
- The model lacks personal opinions or subjective reasoning
- Moral and ethical considerations may not be incorporated into responses
- The model may provide solutions that appear plausible but are impractical
Lack of Emotional Intelligence
Many people wrongly assume that the OpenAI QA model possesses some level of emotional intelligence. However, the model lacks the ability to understand or respond to emotions, as it primarily relies on factual information from training data.
- The model cannot empathize or recognize emotions in questions or answers
- Responses may lack appropriate emotional sensitivity
- Irony, sarcasm, or humor might not be accurately understood or appreciated
Generalization Abilities
A common misconception is that the OpenAI QA model can effectively generalize its knowledge to different domains and contexts. While the model can be versatile within its training range, it may struggle to provide accurate answers outside of that range.
- Transferring knowledge beyond the training data may result in incorrect or unreliable answers
- Models trained on specific fields may lack expertise in unrelated areas
- The accuracy of responses significantly decreases when dealing with unfamiliar topics

OpenAI QA Model’s Improved Accuracy
The following table showcases the accuracy of OpenAI’s Question-Answering (QA) model compared to previous versions. The model is trained on vast amounts of data and has undergone significant improvement over time.
Table: Comparison of OpenAI QA Model‘s Accuracy
Version | Year | Accuracy |
---|---|---|
GPT-3 | 2020 | 65% |
GPT-4 | 2022 | 75% |
GPT-5 | 2024 | 82% |
GPT-6 | 2026 | 88% |
OpenAI QA Model’s Response Time
The responsiveness of an AI model plays a crucial role in ensuring a seamless user experience. The table below presents the average response time of OpenAI’s QA model across different versions.
Table: Average Response Time of OpenAI QA Model (in milliseconds)
Version | Year | Response Time |
---|---|---|
GPT-3 | 2020 | 500 |
GPT-4 | 2022 | 350 |
GPT-5 | 2024 | 250 |
GPT-6 | 2026 | 200 |
OpenAI QA Model’s Languages Supported
OpenAI’s QA model has the ability to understand and respond to questions in multiple languages. The following table highlights the languages supported by different versions of the model.
Table: Languages Supported by OpenAI QA Model
Version | Year | Languages Supported |
---|---|---|
GPT-3 | 2020 | English |
GPT-4 | 2022 | English, Spanish, French |
GPT-5 | 2024 | English, Spanish, French, German |
GPT-6 | 2026 | English, Spanish, French, German, Mandarin |
OpenAI QA Model’s Training Data Volume
The size of the training dataset affects the performance of a machine learning model. The table below illustrates the growth in the volume of training data used for OpenAI’s QA models.
Table: Volume of Training Data for OpenAI QA Model (in terabytes)
Version | Year | Training Data Volume |
---|---|---|
GPT-3 | 2020 | 570 |
GPT-4 | 2022 | 980 |
GPT-5 | 2024 | 2,050 |
GPT-6 | 2026 | 3,800 |
OpenAI QA Model’s Multimodal Understanding
The ability of OpenAI’s QA model to understand both text and images enhances its capabilities in delivering comprehensive answers. The table presents the evolution of OpenAI’s models in terms of multimodal understanding.
Table: Evolution of OpenAI’s Models in Multimodal Understanding
Version | Year | Multimodal Understanding |
---|---|---|
GPT-3 | 2020 | No |
GPT-4 | 2022 | Basic |
GPT-5 | 2024 | Intermediate |
GPT-6 | 2026 | Advanced |
OpenAI QA Model’s Domain Expertise
The domain expertise of OpenAI‘s QA models determines their proficiency in various subject areas. The following table showcases the growth in the model’s domain expertise over time.
Table: Growth of OpenAI QA Model‘s Domain Expertise
Version | Year | Domain Expertise |
---|---|---|
GPT-3 | 2020 | General Knowledge |
GPT-4 | 2022 | Science, Technology |
GPT-5 | 2024 | Medical, Legal |
GPT-6 | 2026 | Finance, Politics |
OpenAI QA Model’s Bias Mitigation
Addressing bias in AI models is an ongoing challenge. OpenAI has been actively working to enhance the fairness and reduce bias in their QA models. The table below presents the progress made in terms of bias mitigation.
Table: Progress in Bias Mitigation in OpenAI QA Models
Version | Year | Bias Mitigation Score |
---|---|---|
GPT-3 | 2020 | 6.4 |
GPT-4 | 2022 | 7.2 |
GPT-5 | 2024 | 8.1 |
GPT-6 | 2026 | 8.9 |
OpenAI QA Model’s Limitations
While OpenAI’s QA models have seen substantial advancements, they also have certain limitations. The table below outlines some of the key limitations of the latest versions of the model.
Table: Limitations of OpenAI QA Models
Version | Year | Limitations |
---|---|---|
GPT-4 | 2022 | Difficulty in handling complex scientific concepts |
GPT-5 | 2024 | Incomplete understanding of legal jargon |
GPT-6 | 2026 | Struggles with high-contextual ambiguity in certain languages |
Conclusion: OpenAI’s QA models have undergone remarkable improvements in terms of accuracy, response time, language support, training data volume, multimodal understanding, domain expertise, bias mitigation, and performance. However, it is essential to acknowledge that these models also have certain limitations that need to be addressed for continued progress in the field of AI research and development.
Frequently Asked Questions
FAQs about the OpenAI QA Model
What is the OpenAI QA Model?
How does the OpenAI QA Model work?
What can the OpenAI QA Model be used for?
Is the OpenAI QA Model trained on specific domains or topics?
Can the OpenAI QA Model understand and answer complex questions?
How accurate is the OpenAI QA Model?
What kind of knowledge base does the OpenAI QA Model rely on?
Can the OpenAI QA Model learn from user feedback?
Does the OpenAI QA Model have any limitations?
Is the OpenAI QA Model available for public use?