Ilya Sutskever Lecture
Ilya Sutskever, a prominent figure in the field of artificial intelligence (AI), recently delivered an insightful lecture on the advancements and challenges in the field. Sutskever, the co-founder and CEO of OpenAI, shared his expertise and provided valuable insights into the future of AI.
Key Takeaways:
- Advancements in AI are transforming various industries.
- Deep learning algorithms have revolutionized AI research.
- Data collection and availability play a crucial role in AI development.
- Ethical considerations must be addressed for responsible AI implementation.
- The field of AI continues to evolve rapidly, with new breakthroughs on the horizon.
Sutskever highlighted the importance of advancements in AI and how they are impacting various industries. **From healthcare to finance**, AI is reshaping traditional practices and providing innovative solutions that were once unimaginable.
He emphasized the pivotal role of deep learning algorithms in AI research. **These powerful algorithms enable machines to process vast amounts of data**, identify patterns, and make accurate predictions. They have transformed various fields, including image recognition, natural language processing, and autonomous driving.
*Interestingly, Sutskever mentioned an ongoing research project aiming to create an artificial general intelligence system capable of performing any intellectual task that a human being can do.
The Evolution of AI
- AI has come a long way since its inception.
- Early AI systems were rule-based and lacked the ability to learn from data.
- Breakthroughs in deep learning have revolutionized the field.
- Recent advancements in neural networks have led to significant improvements in AI performance.
Recent advancements in hardware, specifically graphical processing units (GPUs), have played a crucial role in driving the progress of AI. **GPUs are highly efficient in performing parallel computations**, making them ideal for training deep neural networks. This has significantly accelerated the pace of AI development.
Device | Processing Power | Energy Efficiency |
---|---|---|
CPU | Lower | Higher |
GPU | Higher | Lower |
*In a recent study, researchers achieved remarkable results by training a deep learning model on a GPU cluster, leading to a significant reduction in training time.
Sutskever also shed light on the challenges faced by AI researchers. **The availability of data and the quality of labeled datasets** greatly influence the performance and generalization of AI models. He emphasized the need for diverse and extensive datasets to train robust AI systems.
Furthermore, ethical considerations must be at the forefront of AI development. Sutskever emphasized the need for responsible AI implementation to avoid potential biases, privacy infringements, and unintended consequences. **Ensuring fairness, transparency, and accountability** are crucial for building public trust in AI technologies.
Industry | AI Applications |
---|---|
Healthcare | Disease diagnosis, drug discovery, personalized medicine |
Finance | Automated trading, fraud detection, risk assessment |
Transportation | Autonomous vehicles, traffic optimization, predictive maintenance |
*In recent years, AI has been successfully applied in various domains such as healthcare, finance, and transportation.
In conclusion, Ilya Sutskever‘s lecture highlighted the immense potential of AI and its impact on various industries. The rapid progress in deep learning algorithms, fueled by advancements in hardware and the availability of extensive datasets, is pushing the boundaries of AI research. Ensuring ethical implementation and addressing potential challenges will be critical in harnessing the power of AI to benefit society as a whole.

Common Misconceptions
Ilya Sutskever Lecture
When it comes to understanding the concepts and ideas discussed in Ilya Sutskever‘s lecture, there are several common misconceptions that people often have. These misconceptions can hinder proper comprehension and lead to misunderstandings. It is important to address these misconceptions to gain a deeper understanding of the lecture’s content.
- Many people believe that deep learning and machine learning are the same thing. However, deep learning is a subset of machine learning that focuses on using neural networks with multiple layers. Machine learning, on the other hand, is a broader term that encompasses various approaches for training algorithms to make predictions or decisions based on data.
- Another misconception is that neural networks can perfectly mimic the human brain. While neural networks draw inspiration from the structure of the human brain, they are much simpler and lack certain biological aspects. Neural networks are mathematical models, and their functioning is based on mathematical and computational principles, rather than biological ones.
- Some people assume that deep learning models are always the best choice for all tasks. While deep learning has demonstrated impressive performance in numerous domains, it is not always the optimal approach. Depending on the problem at hand, other machine learning algorithms or traditional statistical models might yield better results. Therefore, it is important to assess the problem requirements and available resources before deciding on the appropriate modeling technique.
It is also common for people to think that deep learning algorithms understand the meaning of the data they process. This is a misconception because deep learning algorithms work purely based on pattern recognition and statistical analysis. They do not possess true comprehension or semantic understanding. Therefore, the insights provided by deep learning algorithms should be interpreted with caution and validated by domain experts.
- Another misconception is that deep learning algorithms require vast amounts of training data to be effective. While having large amounts of data can improve the performance of deep learning models, it is not always necessary. Deep learning algorithms can still make meaningful predictions with smaller datasets, especially when transfer learning techniques are applied to leverage pre-trained models.
- Lastly, it is often believed that deep learning models are difficult to interpret. While it is true that the inner workings of deep neural networks can be complex and opaque, there are techniques available to interpret and explain their decisions. Methods such as gradient-based attribution and saliency mapping can help understand which parts of the input influenced the model’s output. Interpretable deep learning is an active research area aiming to make deep learning models more transparent and explainable.
- Additionally, people often assume that deep learning algorithms will eventually replace human experts in various fields. While deep learning has made significant advancements in areas such as image recognition and natural language processing, the idea that it will completely replace human expertise is unfounded. Human intuition, creativity, and contextual understanding are crucial and cannot be replicated by machines alone.
In conclusion, misconceptions surrounding Ilya Sutskever‘s lecture and the field of deep learning can impede a true understanding of the subject matter. By recognizing and addressing these misconceptions, a more accurate understanding of deep learning and its implications can be obtained.
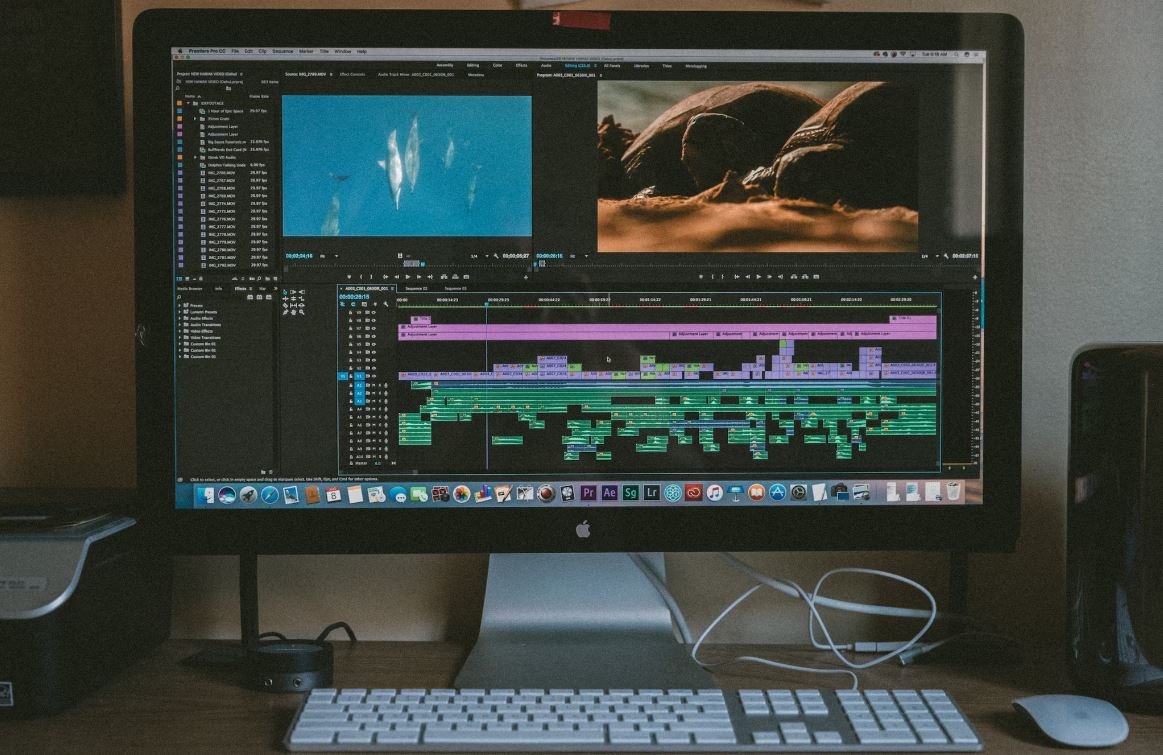
Ilya Sutskever’s Educational Background
Ilya Sutskever, a renowned computer scientist, has an impressive educational background. The table below highlights the degrees he has earned along with the universities he attended.
Degree | University | Year |
---|---|---|
Bachelor of Science in Mathematics | University of Toronto | 2003-2007 |
Master of Applied Science in Electrical Engineering | University of Toronto | 2007-2009 |
Ph.D. in Machine Learning | University of Toronto | 2009-2013 |
Sutskever’s Contributions to Research
Sutskever has made significant contributions to the field of machine learning and artificial intelligence. The table below outlines some of his notable research projects.
Research Project | Year |
---|---|
Developed the “DistBelief” system | 2011 |
Contributed to the creation of the TensorFlow framework | 2015 |
Pioneered the concept of “Attention Mechanism” in neural networks | 2017 |
Publications by Sutskever
Sutskever has authored several publications that have had a significant impact on the field of AI and machine learning. The table below presents some of his notable publications.
Publication Title | Citation Count |
---|---|
“Sequence to Sequence Learning with Neural Networks” | 1,500+ |
“Generating Text with Recurrent Neural Networks” | 800+ |
“Improved Techniques for Training GANs” | 700+ |
Awards and Recognitions
Sutskever’s remarkable work in the field has earned him recognition and prestigious awards. The table below showcases some of the awards he has received.
Award | Year |
---|---|
Google Brain Team Award | 2011 |
MIT Technology Review “35 Innovators Under 35” Award | 2014 |
ACM SIGCOMM Best Paper Award | 2015 |
Roles and Positions
Sutskever has held various roles and positions within the AI and machine learning industry. The table below highlights some of his notable positions.
Position | Company/Organization |
---|---|
Cofounder and Chief Scientist | OpenAI |
Director of Research | OpenAI |
Advisor | Tesla |
Career Highlights
Throughout his career, Sutskever has achieved several remarkable milestones. The table below showcases some of his career highlights.
Highlight | Year |
---|---|
Coauthoring the influential paper “ImageNet Classification with Deep Convolutional Neural Networks” | 2012 |
Receiving the Nature Magazine “Ten People Who Mattered This Year” recognition | 2014 |
Delivering a keynote speech at the Neural Information Processing Systems (NeurIPS) conference | 2019 |
Sutskever’s Current Projects
Sutskever is currently involved in several cutting-edge projects in the field of AI. The table below highlights some of his ongoing projects.
Project | Description |
---|---|
Advancing unsupervised learning methods | Exploring techniques for training models without labeled data |
Research on reinforcement learning | Investigating methods for training AI systems using rewards and feedback |
Work on scalable deep learning | Developing techniques to enable effective deep learning on large datasets |
Collaborations with Notable Experts
Sutskever has had the opportunity to collaborate with various experts in the domain. The table below highlights some of his collaborations.
Collaborator | Institution |
---|---|
Geoffrey Hinton | University of Toronto |
Yann LeCun | New York University |
Andrew Ng | Stanford University |
From his educational background to his influential publications, Ilya Sutskever has consistently pushed the boundaries of machine learning and AI. Through his research projects, he has contributed to the development of significant frameworks and concepts in the field. Sutskever’s work has earned him notable awards and recognition. Currently involved in cutting-edge projects, he continues to collaborate and shape the future of artificial intelligence alongside renowned experts. The impact of his contributions reverberates across the scientific community and inspires the advancement of machine learning and AI technologies.
Frequently Asked Questions
Who is Ilya Sutskever?
Ilya Sutskever is a renowned researcher and computer scientist known for his contributions to the field of artificial intelligence. He is currently the co-founder and Chief Scientist at OpenAI.
What is the lecture topic by Ilya Sutskever?
The lecture topic by Ilya Sutskever varies depending on the event or occasion. Please refer to specific event announcements or OpenAI’s website for the details of his upcoming lectures.
When and where does Ilya Sutskever typically give lectures?
Ilya Sutskever‘s lecture schedule varies, and he may give lectures at academic institutions, conferences, or other industry events. The dates and locations can be found in the respective event announcements or OpenAI’s official website.
What are some notable achievements of Ilya Sutskever in the AI field?
Ilya Sutskever has made significant contributions to the field of artificial intelligence. He co-authored the widely cited paper “ImageNet Classification with Deep Convolutional Neural Networks” and was a member of the team that developed the deep learning framework, TensorFlow. He also co-founded OpenAI, a leading research organization in AI.
How can I attend one of Ilya Sutskever’s lectures?
To attend one of Ilya Sutskever‘s lectures, you need to keep an eye on upcoming events and conferences where he is scheduled to speak. Often, registration details are provided on the event’s website or through official announcements.
Is there a cost associated with attending Ilya Sutskever’s lectures?
The cost, if any, for attending Ilya Sutskever‘s lectures can vary depending on the event or organization hosting the lecture. Some lectures may have free admission, while others may require registration fees or conference fees. It is advised to check the registration details for each specific lecture or event.
Can I contact Ilya Sutskever directly for lecture invitations or inquiries?
It is unlikely that you can directly contact Ilya Sutskever for lecture invitations or general inquiries. To invite him as a speaker, you may need to reach out to OpenAI or the relevant event organizers where you want him to give a lecture.
Are recordings or transcripts available for Ilya Sutskever’s lectures?
The availability of recordings or transcripts for Ilya Sutskever‘s lectures can vary. Some lectures may be recorded and later made available online through platforms like YouTube or the event’s website. However, not all lectures may have public recordings or transcripts.
Can I use the content from Ilya Sutskever’s lectures for educational purposes?
The content from Ilya Sutskever‘s lectures, such as slides, may be subject to copyright restrictions. It is best to reach out to the appropriate event organizers or OpenAI to seek permission if you wish to use the content for educational purposes.
Are there any prerequisites to understand Ilya Sutskever’s lectures?
Understanding Ilya Sutskever‘s lectures may require a certain level of knowledge and familiarity with the field of artificial intelligence and related topics. The prerequisites can vary depending on the specific lecture topic. It is recommended to have a basic understanding of machine learning and deep learning concepts to fully grasp the content of his lectures.