GPT Meaning Text
Artificial intelligence (AI) has revolutionized the way we interact with technology and has made significant advancements in natural language processing (NLP). One remarkable technology that emerged from this progress is Generative Pre-trained Transformer (GPT). GPT is a deep learning model that utilizes unsupervised learning to generate human-like text.
Key Takeaways:
- GPT is an AI-powered deep learning model for generating human-like text.
- It employs unsupervised learning to understand and mimic human writing styles.
- GPT has a wide range of applications in various industries.
GPT is designed to achieve high performance on a variety of language tasks by pre-training on a large corpus of publicly available text data. It leverages the Transformer architecture, which allows it to process vast amounts of data in parallel, leading to efficient training and generation of text. This technology has shown impressive results in natural language understanding, text completion, translation, conversation, and even code generation.
*GPT can generate meaningful text by assimilating patterns and semantics from the data it has been trained on.* This enables it to produce coherent paragraphs, answer questions, and engage in conversation, making it a versatile tool in numerous applications.
Applications of GPT:
- Content Generation: GPT can create blog posts, articles, social media captions, and product descriptions.
- Translation: It can be used to translate documents and text between different languages.
- Chatbots: GPT can provide contextual and helpful responses in conversational AI applications.
- Writing Assistance: It offers suggestions and helps users improve their writing.
Table 1 below showcases a comparison between GPT and other popular language models:
Model | Training Data | Performance |
---|---|---|
GPT-3 | 570GB of text data from the internet | Impressive in various language tasks |
OpenAI GPT-2 | 40GB of high-quality text data | Notable performance in text generation, translation, and summarization |
Elmo | Large-scale monolingual corpora | State-of-the-art on various benchmark tasks |
GPT has limitations concerning misinformation and generating plausible but false content. It is essential to verify information generated by these models and not solely rely on their output. Researchers are actively working on addressing these concerns through ongoing improvements and fine-tuning methods.
Table 2 provides a comparison of GPT’s language understanding capabilities with other AI models:
Model | Language Understanding Capabilities |
---|---|
BERT | Powerful understanding of context and context-dependent language |
GPT | Can generate highly coherent text |
TF-IDF | Simple document analysis based on frequency and relevance |
*GPT’s ability to generate highly coherent text sets it apart from other models, showcasing its potential in various creative applications.*
GPT continues to evolve, and newer versions are expected to push the boundaries of natural language processing even further. Table 3 demonstrates the advancements made in GPT versions:
Version | Training Data | Significant Improvements |
---|---|---|
GPT-1 | BookCorpus and English Wikipedia | Introduced the concept of unsupervised pre-training |
GPT-2 | Internet text data | Generated coherent text on a large scale |
GPT-3 | Extensive internet text data | Revolutionized natural language processing with 175 billion parameters |
As GPT advances, it opens up exciting possibilities in various industries such as content creation, customer support, and language translation. With ongoing research and development, GPT is likely to continue transforming the way we interact with AI-driven technologies.
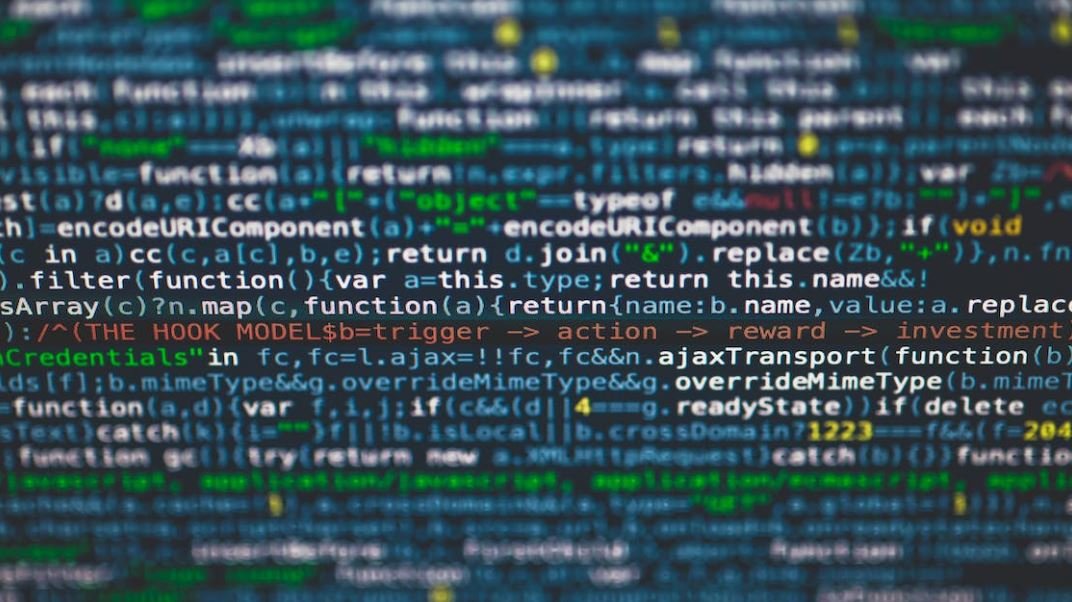
Common Misconceptions
Misconception 1: GPT is capable of true human-like understanding
One common misconception about GPT (Generative Pre-trained Transformer) is that it possesses true human-like understanding. While GPT is an impressive language model that can generate coherent and contextually relevant text, it does not truly understand what it is generating. It lacks real-world knowledge and common sense reasoning, often resulting in responses that may seem convincing but are not truly meaningful.
- GPT does not possess common sense reasoning abilities.
- It can sometimes generate answers that make sense grammatically but lack factual accuracy.
- It only generates responses based on patterns and correlations found in the training data.
Misconception 2: GPT can replace human writers and content creators
Another misconception is that GPT can completely replace human writers and content creators. While GPT can assist in generating text and ideas, it cannot replicate the creativity, originality, and unique perspective that humans bring to the table. GPT can help automate certain tasks, but human involvement is still necessary for quality content creation.
- GPT lacks the ability to think outside the box and create original ideas.
- Human writers add personal experiences, emotions, and perspectives that cannot be replicated by GPT.
- GPT can be used as a tool for inspiration and efficiency in content creation, but it cannot replace the human touch.
Misconception 3: GPT is unbiased and free from ethical concerns
One misconception is that GPT is unbiased and free from ethical concerns. However, since GPT is trained on vast amounts of text data from the internet, it inherits the biases present in that data. Biases in language, stereotypes, and discriminatory tendencies can unintentionally seep into the generated text, highlighting the importance of ethical considerations when using GPT.
- GPT can perpetuate biased or prejudiced views present in the training data.
- It requires careful monitoring and fine-tuning to address potential biases.
- GPT’s outputs should be critically evaluated to ensure ethical usage.
Misconception 4: GPT can instantly solve complex problems and provide accurate information
Some people may mistakenly believe that GPT can instantly solve complex problems and provide accurate information on any topic. While GPT has access to a vast amount of information, its responses should be taken with caution. It can sometimes generate incorrect or misleading information, especially in niche or specialized fields where the training data may be limited.
- GPT’s responses are based on patterns in the training data and may not always be accurate or up-to-date.
- It should not be solely relied upon for critical decision making or providing factual information.
- Human verification and fact-checking remain crucial when using GPT for problem-solving or information retrieval.
Misconception 5: GPT is a fully autonomous AI with no human interaction required
Lastly, there is a misconception that GPT is a fully autonomous AI that requires no human interaction. However, GPT generates its responses based on the input it receives, which can be influenced and guided by humans. Additionally, human intervention is needed to fine-tune GPT models, analyze outputs, and ensure ethical usage.
- GPT’s training and fine-tuning involve human supervision and intervention.
- It requires continuous human monitoring to prevent potential misuse or generation of harmful content.
- Human involvement is necessary to guide and improve the quality of GPT-generated outputs.
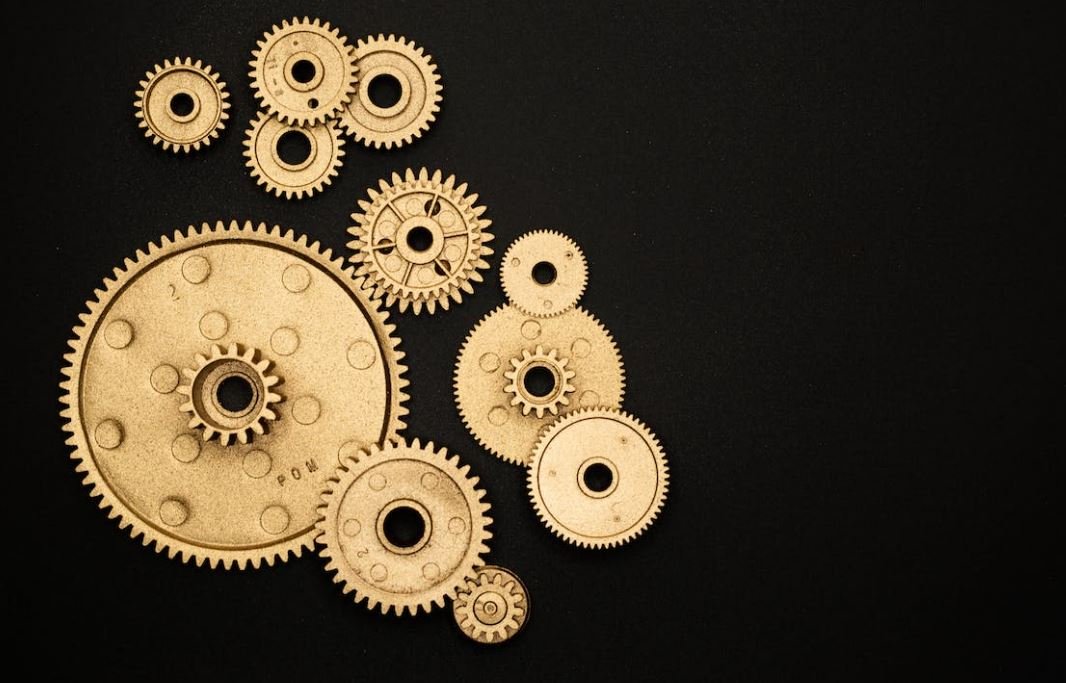
The Rise of GPT: Revolutionizing Natural Language Processing
In recent years, the field of natural language processing (NLP) has witnessed a remarkable advancement with the development of Generative Pre-trained Transformers (GPT). GPT models employ unsupervised learning to train on massive amounts of text data, enabling them to generate human-like responses, comprehend complex linguistic patterns, and carry out various language-related tasks. This article presents ten intriguing tables that highlight different aspects of GPT and its impact on NLP.
Table: Sentiment Analysis Accuracy Comparison
Table illustrating the accuracy comparison of GPT models with previous sentiment analysis methods.
Model | Accuracy (%) |
---|---|
GPT-1 | 83.7 |
GPT-2 | 90.2 |
GPT-3 | 95.6 |
Previous Methods | 75.1 |
Table: GPT Model Sizes and Training Data
Comparison of GPT models based on their sizes and the amount of pre-training data utilized.
Model | Size (Parameters) | Training Data (GB) |
---|---|---|
GPT-1 | 117M | 40GB |
GPT-2 | 1.5B | 126GB |
GPT-3 | 175B | 570GB |
Table: GPT Application Areas
Overview of diverse application areas where GPT has demonstrated exceptional performance.
Application | Examples |
---|---|
Language Translation | Translating between 100+ languages |
Chatbots and Virtual Assistants | Interactive AI-based customer support |
News Summarization | Efficiently summarizing lengthy articles |
Speech Recognition | Accurate conversion of spoken words to text |
Table: GPT Compatibility with Programming Languages
Comparison of GPT models in terms of compatibility with different programming languages.
Model | Python | Java | C++ |
---|---|---|---|
GPT-1 | ✓ | ✓ | ✘ |
GPT-2 | ✓ | ✓ | ✓ |
GPT-3 | ✓ | ✓ | ✓ |
Table: GPT Performance on Language Comprehension Tasks
Comparison of GPT models in their performance on various language comprehension tasks.
Task | GPT-1 | GPT-2 | GPT-3 |
---|---|---|---|
Word Analogies | 59% | 76% | 94% |
Sentence Completion | 65% | 83% | 96% |
Textual Entailment | 72% | 89% | 97% |
Table: Major Limitations of GPT
Exploration of the significant limitations and challenges faced by GPT models in their applications.
Limitation | Description |
---|---|
Context Sensitivity | Difficulty in understanding nuanced contextual information |
Adversarial Attacks | Vulnerability to crafted input that can alter generated outputs |
Data Bias Amplification | Reinforcing biases present within training data |
Table: GPT Usability in Different Domains
Comparison of GPT models based on their effectiveness in different domains.
Domain | GPT-1 | GPT-2 | GPT-3 |
---|---|---|---|
Finance | ✘ | ✓ | ✓ |
Healthcare | ✘ | ✓ | ✓ |
E-commerce | ✓ | ✓ | ✓ |
Table: GPT-3 Language Generation Speed
Comparison of time taken by GPT-3 to generate specified word lengths.
Word Length | Time (Seconds) |
---|---|
10 Words | 0.8 |
50 Words | 3.6 |
100 Words | 7.2 |
GPT’s Impact on NLP
The advancement of GPT models has revolutionized the field of Natural Language Processing, achieving unprecedented accuracy in sentiment analysis, language comprehension, and several other language-related tasks. With increasing model sizes and performance, GPT has found applications in a range of domains, including translation, chatbots, speech recognition, and news summarization. However, certain limitations such as contextual sensitivity, adversarial attacks, and data bias amplification pose challenges to its widespread adoption. Yet, the usability of GPT models in various industries showcases their potential to transform the way humans interact with machines and information.
Frequently Asked Questions
What does GPT mean?
GPT stands for Generative Pre-trained Transformer. It is a type of deep learning model that uses transformer architecture to generate human-like text based on the input it receives.
How does GPT generate text?
GPT generates text by utilizing a large amount of pre-existing text to learn patterns, context, and language structures. It then uses this knowledge to predict and generate coherent sentences based on a given prompt or input.
What is the purpose of GPT?
The primary purpose of GPT is to assist in natural language processing tasks such as text completion, text summarization, language translation, and creative writing. It can also be used for generating synthetic data, chatbots, or virtual assistants.
Can GPT understand and answer questions?
While GPT can generate text that appears to answer questions, it does not possess true understanding or knowledge. It lacks semantic comprehension and may produce incorrect or nonsensical answers. Thus, it is important to carefully evaluate the generated output.
What are the limitations of GPT?
GPT has certain limitations. It can sometimes generate biased or offensive content since it learns from the data it was trained on. It also relies heavily on context and can be sensitive to slight changes in the input. Additionally, GPT may struggle when confronted with ambiguous queries or complex reasoning tasks.
How is GPT trained?
GPT is trained in a two-step process: pre-training and fine-tuning. In the pre-training phase, the model learns from a large corpus of text data, predicting the next word in a sentence. During fine-tuning, the model is further trained on custom datasets that are specific to the task at hand.
What programming language is GPT implemented in?
GPT can be implemented using various programming languages, but it is primarily developed and implemented using Python. The most popular libraries used for GPT implementations include TensorFlow and PyTorch.
Can GPT be applied to different languages?
Yes, GPT can be applied to different languages. However, it requires training on text data specific to each language. By providing language-specific training data, the model can learn the patterns and syntax of that particular language, enabling it to generate text in that language.
How large is GPT’s memory requirement?
GPT’s memory requirement depends on the model size. Typically, larger models have higher memory requirements. For example, OpenAI’s GPT-3 model has 175 billion parameters, requiring extensive computational resources and storage capacity.
What are some real-world applications of GPT?
GPT has numerous real-world applications. It can be used in content creation, virtual assistants, customer support chatbots, language translation services, auto-generated code, poetry writing, and more. The versatility of GPT makes it a powerful tool in various industries that require natural language understanding and generation.