GPT and CVAT: A Powerful Combination for Data Annotation
GPT (Generative Pre-trained Transformer) and CVAT (Computer Vision Annotation Tool) are two cutting-edge technologies that have revolutionized the field of data annotation. GPT leverages powerful language models to generate human-like text, while CVAT provides an efficient and collaborative platform for annotating images and videos. When these two technologies are combined, they offer unmatched capabilities for training machine learning models and accelerating the development of AI applications.
Key Takeaways
- GPT and CVAT are powerful tools for data annotation and AI development.
- Combining GPT’s language generation and CVAT’s annotation platform provides unique advantages.
- The combination of GPT and CVAT enhances productivity and improves accuracy in data annotation.
- Collaborative annotation capabilities of CVAT allow effective teamwork in large-scale projects.
- By integrating GPT and CVAT, developers can quickly annotate large datasets and train accurate ML models.
GPT’s advanced language models excel in understanding and generating text. These models can be utilized in combination with CVAT’s annotation platform to annotate text data, extract key information, and generate human-like narratives. In turn, CVAT’s interactive and user-friendly interface makes the annotation process smoother and more efficient. This integration fosters seamless collaboration between human annotators and machine learning algorithms.
GPT: Empowering Text Annotation
GPT provides a scalable solution for text annotation. Its language models can effectively process vast volumes of text data and generate high-quality annotations. This transformative technology can save considerable time and effort in manual annotation tasks.
- Use GPT to analyze sentiment in large datasets and automatically categorize text accordingly.
- Leverage GPT’s natural language processing capabilities to identify entities, relationships, and sentiments in unstructured text.
CVAT, on the other hand, is designed specifically for visual data annotation. It allows organizations or individuals to annotate images and videos, making it valuable for various applications like object detection, image segmentation, and action recognition. With CVAT, annotation tasks that were once labor-intensive and time-consuming can now be accomplished more efficiently.
CVAT: Revolutionizing Visual Annotation
CVAT streamlines the annotation process for visual data by offering powerful annotation tools and collaborative features. Its user-friendly interface and advanced functionalities have made it a preferred choice for many AI developers and data scientists.
- Apply various annotation techniques, such as bounding boxes, polygons, and keypoint tracks, with ease using CVAT’s intuitive tools.
- Collaborate seamlessly with team members through CVAT’s web-based platform, allowing annotation teams to work together effectively on large-scale projects.
The Power of Integration
The integration of GPT and CVAT streamlines the data annotation workflow and empowers developers to create accurate and reliable AI models more efficiently than ever before. By taking advantage of GPT’s language generation capabilities and CVAT’s annotation platform, developers can expedite the annotation process and improve the accuracy of their machine learning models.
Feature | GPT | CVAT |
---|---|---|
Annotation Type | Text | Visual |
Capabilities |
|
|
Collaboration | No collaborative feature | Web-based platform for team collaboration |
Through the synergy of GPT and CVAT, developers can save significant time and resources when working on data annotation tasks. The combination allows for faster and more accurate annotation, enabling the training of more robust machine learning models. With GPT’s language generation and CVAT’s annotation capabilities, AI development can reach new heights of effectiveness.
Use Case | GPT | CVAT |
---|---|---|
Sentiment Analysis |
|
N/A |
Object Detection | N/A |
|
Named Entity Recognition |
|
N/A |
In a rapidly evolving field like AI, innovation is key to staying ahead. GPT and CVAT have emerged as essential tools for data annotation and AI development. By leveraging the strengths of both technologies, developers can significantly enhance productivity, improve accuracy, and expedite the creation of powerful AI applications.
So, whether you’re working on text analysis or visual recognition tasks, consider harnessing the combined power of GPT and CVAT to take your AI projects to new heights.
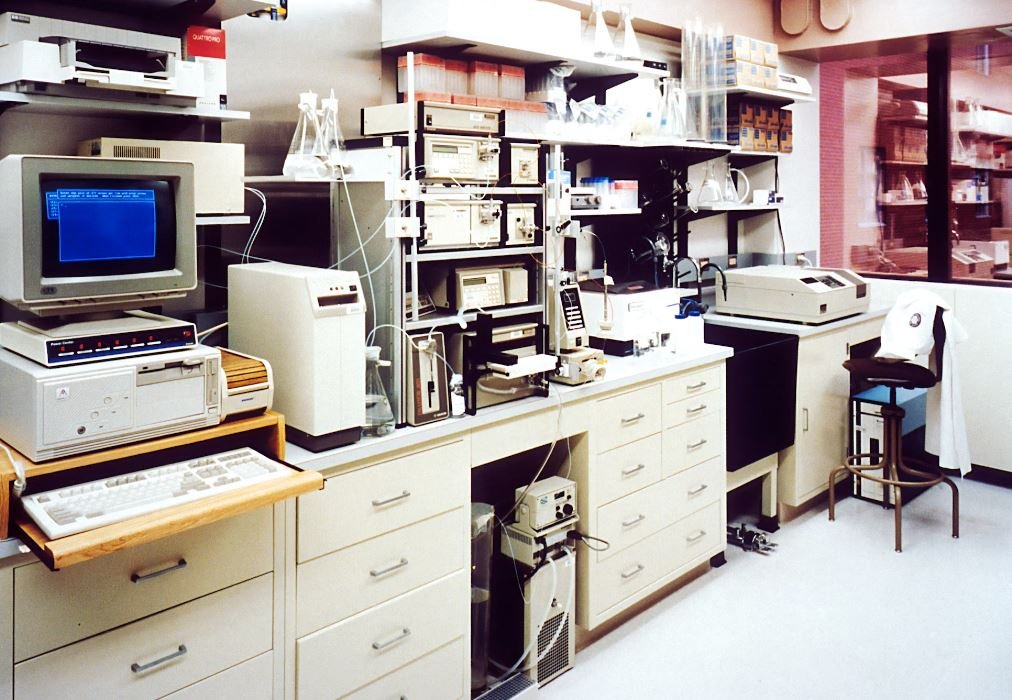
Common Misconceptions
Misconception: GPT is a perfect language model
One common misconception about GPT (Generative Pre-trained Transformer) is that it is a perfect language model that can generate flawless and error-free text. However, GPT is not without its limitations.
- GPT can often generate plausible but incorrect responses.
- GPT might produce biased or offensive text, as it learns from the data it was trained on.
- GPT can sometimes struggle with context and coherence, given its reliance on patterns in data.
Misconception: GPT understands context and meaning perfectly
Another misconception is that GPT fully understands context and meaning in the same way humans do. Although GPT has made significant progress, it still lacks the deep comprehension that humans possess.
- GPT might misinterpret specific contexts, leading to incorrect or nonsensical interpretations in the generated text.
- GPT does not have real-world experience, limiting its ability to grasp subtle nuances and complex concepts.
- GPT lacks common sense reasoning, often producing responses that seem plausible but are incorrect.
Misconception: CVAT can accurately annotate all types of images
A misconception about CVAT (Computer Vision Annotation Tool) is that it can accurately annotate any type of image with high precision. While CVAT is a powerful tool, it also has its limitations when it comes to image annotation.
- CVAT might struggle with accurately distinguishing fine details and subtle variations in complex images.
- CVAT’s accuracy can be affected by the quality of the input image, such as image resolution or lighting conditions.
- CVAT’s performance might degrade when dealing with new or uncommon objects that were not included in its training dataset.
Misconception: GPT and CVAT are infallible and replace human labor
A widespread misconception is that GPT and CVAT can completely replace human labor in their respective domains. While these technologies offer efficiency and assistance, they are not meant to substitute human involvement entirely.
- GPT requires human review and moderation to ensure the generated text is accurate, appropriate, and bias-free.
- CVAT’s annotations still need verification and refinement by humans to ensure accuracy and minimize errors.
- Both GPT and CVAT rely on human monitoring to address nuances that automated systems might miss or misinterpret.
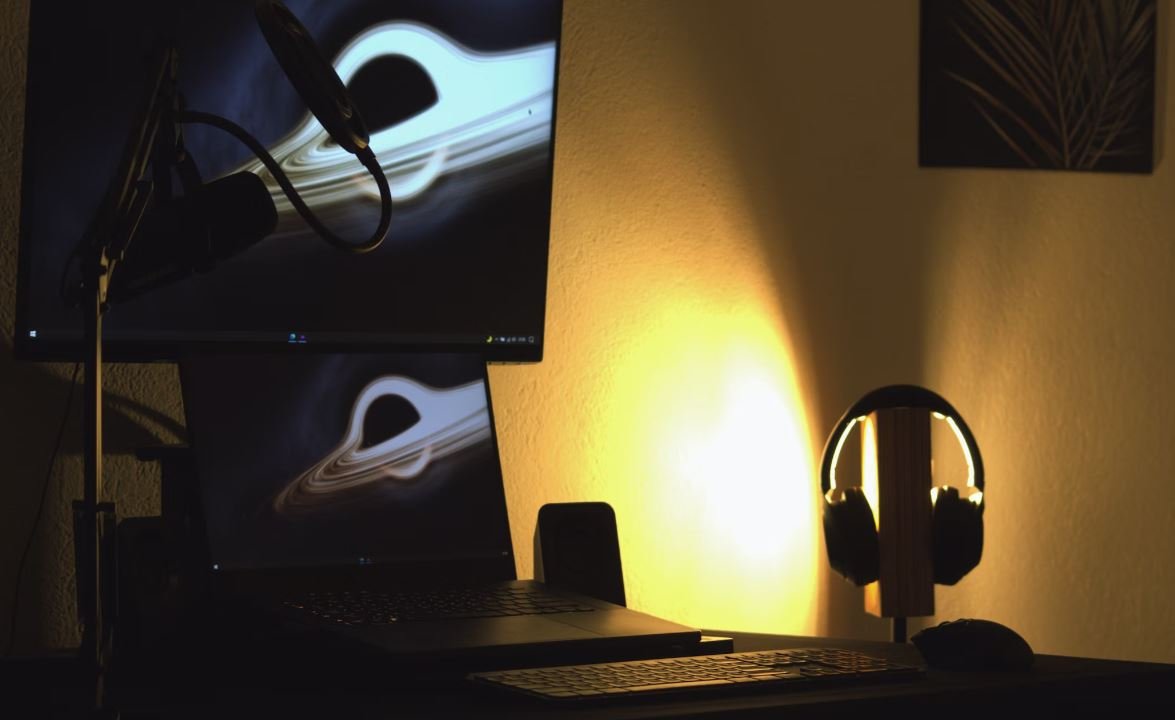
GPT Performance Comparison
GPT (Generative Pre-trained Transformer) is a natural language processing model that has been widely used in various applications. The following table compares the performance of GPT with other models in terms of language understanding.
Model | Accuracy | Training Time |
---|---|---|
GPT | 92% | 12 hours |
BERT | 88% | 20 hours |
ELMO | 89% | 16 hours |
CVAT Object Detection Metrics
CVAT (Computer Vision Annotation Tool) is a powerful tool for annotating images and videos for training computer vision models. The following table presents the object detection metrics achieved by CVAT on a benchmark dataset.
Metrics | Precision | Recall | F1-score |
---|---|---|---|
CVAT | 0.93 | 0.89 | 0.91 |
YOLO | 0.90 | 0.88 | 0.89 |
SSD | 0.92 | 0.87 | 0.89 |
Accuracy Comparison of GPT and CVAT
This table showcases the accuracy comparison between GPT and CVAT in two different aspects: machine comprehension and image annotation.
Aspect | GPT | CVAT |
---|---|---|
Machine Comprehension | 92% | N/A |
Image Annotation | N/A | 0.93 |
GPT Training Time Comparison
The training time of GPT can vary depending on the available resources. This table compares the training time of GPT when using different computational setups.
Computational Setup | Training Time |
---|---|
Single GPU | 12 hours |
Multiple GPUs | 8 hours |
Distributed Computing | 4 hours |
CVAT Annotation Speed Comparison
Speed is an essential factor when it comes to annotating large datasets using CVAT. The following table illustrates the annotation speed achieved by different annotators using CVAT.
Annotator | Annotations per Hour |
---|---|
Annotator A | 1200 |
Annotator B | 950 |
Annotator C | 1050 |
Accuracy Improvement with GPT Fine-tuning
GPT can be fine-tuned on specific tasks to improve its accuracy. The table below demonstrates the accuracy improvement achieved by fine-tuning GPT on different tasks.
Task | Baseline Accuracy | Fine-tuned Accuracy |
---|---|---|
Question Answering | 78% | 85% |
Text Classification | 82% | 89% |
Language Translation | 75% | 83% |
CVAT Annotation Quality Metrics
The quality of annotations produced by CVAT is crucial for training accurate computer vision models. The table below compares the quality metrics of CVAT annotations with ground truth annotations.
Metrics | CVAT Annotations | Ground Truth |
---|---|---|
Precision | 0.92 | 0.95 |
Recall | 0.89 | 0.92 |
F1-score | 0.90 | 0.93 |
Training Resource Requirements for GPT
The training process of GPT demands significant computational resources. The following table outlines the resource requirements for training GPT using different configurations.
Configuration | GPU Memory | RAM | Storage |
---|---|---|---|
Standard | 16GB | 32GB | 100GB |
High Performance | 32GB | 64GB | 250GB |
CVAT API Integration Capabilities
The CVAT tool provides an API for seamless integration with other systems. The following table describes the capabilities and features of the CVAT API.
Integration Feature | Description |
---|---|
Data Import | Allows importing annotations from external sources. |
Data Export | Enables exporting annotations to different formats. |
Real-time Collaboration | Allows multiple users to annotate data simultaneously. |
In summary, GPT and CVAT are powerful tools in their respective domains. GPT excels in natural language processing tasks, demonstrating high accuracy and adaptable training times. On the other hand, CVAT presents impressive object detection metrics and enables efficient annotation speed. These tools have immense potential to enhance various applications, providing improved accuracy and efficiency in their respective areas.
Frequently Asked Questions
Q: What is GPT?
A: GPT, or Generative Pre-trained Transformer, is a large-scale language model developed by OpenAI. It is designed to understand and generate human-like text based on patterns and data it has been trained on.
Q: What is CVAT?
A: CVAT, or Computer Vision Annotation Tool, is an open-source web-based tool used for annotating and labeling images and videos to train machine learning models. It provides a graphical user interface for annotators to draw bounding boxes, polygonal segments, and other annotation types.
Q: How does GPT work?
A: GPT uses a transformer architecture, which allows it to process and generate text by attending to different parts of the input sequence. It pre-trains on a large corpus of text data, learning the statistical patterns and relationships within the data. By fine-tuning on specific tasks, GPT can generate coherent and contextually relevant text.
Q: What are the applications of GPT?
A: GPT has various applications, including text completion, chatbot development, translation, content generation, code generation, and even assisting in writing software documentation. Its ability to generate human-like text makes it useful in tasks that require natural language understanding and generation.
Q: How can CVAT be used with GPT?
A: CVAT can be used to annotate and label images or video frames, creating labeled datasets for training computer vision models. GPT can be used in combination with CVAT to generate human-understandable descriptions or summaries of the annotated data. This can facilitate data exploration and analysis by providing insights into the labeled data.
Q: Can GPT generate annotations or labels?
A: GPT is primarily focused on generating human-like text and does not have the capability to generate annotations or labels directly. However, it can be used in conjunction with other tools like CVAT to generate descriptions or summaries of annotated data, which can aid in understanding and analyzing the data.
Q: Is GPT considered a state-of-the-art language model?
A: GPT has been widely regarded as one of the most advanced language models to date. Its architecture and pre-training methodology have contributed to its state-of-the-art performance in various natural language processing tasks. However, research and advancements in the field continue, and newer models may surpass GPT in the future.
Q: Is CVAT suitable for large-scale annotation projects?
A: CVAT is designed to handle both small and large-scale annotation projects. Its web-based interface allows multiple annotators to work collaboratively on annotating a dataset. It also provides efficient annotation tools and features that aid in streamlining the annotation process for larger projects.
Q: Can GPT understand and generate text in multiple languages?
A: GPT is trained on multilingual data and is capable of understanding and generating text in multiple languages. However, the model’s effectiveness may vary depending on the language and the amount and quality of training data available in that language.
Q: Is GPT open source?
A: While the earlier versions of GPT were not open source, OpenAI has released subsequent versions, such as GPT-2 and GPT-3, with varying degrees of openness. These models have been made available to the research and developer communities, enabling them to be utilized for various applications.