GPT Engineer
GPT Engineer is an advanced text-generation model by OpenAI that has
revolutionized the field of natural language processing. This powerful AI
system is capable of understanding and generating human-like text across a
wide range of topics and tasks.
Key Takeaways
- GPT Engineer is an advanced text-generation model.
- It can understand and generate human-like text across various domains.
- GPT Engineer has revolutionized natural language processing.
GPT (Generative Pre-trained Transformer) Engineer has gained significant
attention and popularity due to its ability to generate high-quality text
that is coherent and contextually relevant. The model is pre-trained on a
large corpus of text data to learn the underlying patterns and structures
of language, allowing it to generate text with impressive accuracy and
fluency.
GPT Engineer can be utilized in various applications, including content
generation, language translation, chatbots, virtual assistants, and
more. The model can generate articles, product descriptions, dialogues,
and responses to user queries, making it a valuable tool for businesses
and researchers alike.
The secret to GPT Engineer‘s success lies in its unique architecture. It
adopts a transformer-based approach, which enables it to capture and
understand the context of words and sentences. By leveraging its extensive
pre-training, fine-tuning, and the power of deep learning, GPT Engineer
can produce human-like text that is indistinguishable from content written
by humans themselves.
One interesting aspect of GPT Engineer is its ability to engage in
interactive conversations. The model can respond to prompts and questions,
simulating a conversation with a human user. This opens up exciting
possibilities for chatbot applications, virtual assistants, and other
interactive AI systems.
Applications of GPT Engineer
- Content generation for articles, blogs, and websites.
- Language translation and interpretation services.
- Creation of chatbots and virtual assistants.
- Improving search engines and natural language interfaces.
- Data augmentation for machine learning models.
GPT Engineer in Action
To better understand the capabilities of GPT Engineer, let’s take a closer
look at some interesting data points and examples:
Parameter | Value |
---|---|
Size of the model | 1.5 billion parameters |
Training data | Massive amounts of publicly available text |
Language support | Multiple languages including English, Spanish, French, and more |
GPT Engineer has been trained on an extensive dataset consisting of
publicly available text from the internet. This vast training data ensures
that the model has exposure to a wide range of topics and domains,
strengthening its ability to generate coherent and informative text across
different subject matters.
- Table 1 shows the size of the GPT Engineer model, which consists of around 1.5 billion parameters — an indication of its complexity and computational requirements.
- The training data used for GPT Engineer is derived from various sources and encompasses diverse topics, allowing the model to generate text on a wide range of subjects.
- The language support of GPT Engineer extends to multiple languages, facilitating its usability and applicability in various regions and cultures.
Conclusion
GPT Engineer has revolutionized natural language processing, offering
remarkable text-generation capabilities across diverse domains and
languages. Its ability to understand and generate human-like text has made
it an invaluable tool for content creators, businesses, and researchers,
opening up new possibilities for AI applications in the future.
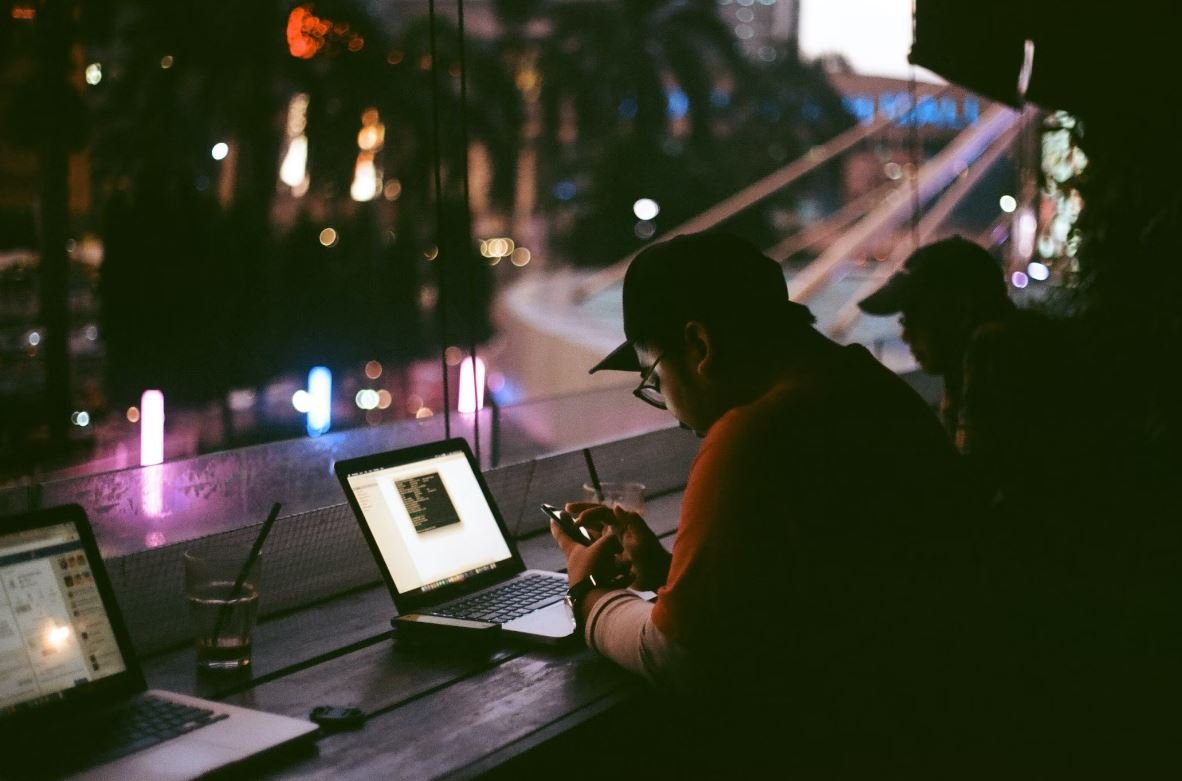
Common Misconceptions
Misconception 1: GPT Engineers Only Focus on Designing Chatbots
One common misconception about GPT Engineers is that their main job is to design chatbots. While chatbot development is one application of GPT (Generative Pre-trained Transformer) technology, GPT Engineers work on a wide range of tasks beyond chatbot development.
- GPT Engineers also work on natural language processing (NLP) projects.
- They develop and improve machine learning models that can understand and generate human-like text.
- GPT Engineers are involved in developing AI models for various industries, including healthcare, finance, and entertainment.
Misconception 2: GPT Engineers Can Automate All Tasks
Another common misconception is that GPT Engineers can automate all tasks using GPT technology. While GPT has remarkable capabilities, there are still limitations to what it can handle.
- GPT Engineers need to carefully curate and train models for specific tasks, which can be time-consuming.
- Some tasks require domain-specific knowledge that GPT might not possess, making automation challenging.
- GPT models benefit from human guidance and feedback to improve their performance and reduce biases.
Misconception 3: GPT Engineers Work Alone
Many people think that GPT Engineers work alone on projects. However, GPT technology is a collaborative effort involving a team of experts from different domains.
- GPT Engineers collaborate with data scientists to collect and preprocess large datasets for training models.
- They work closely with subject-matter experts and domain specialists to understand specific requirements and constraints.
- GPT Engineers also collaborate with software engineers to integrate GPT models into real-world applications.
Misconception 4: GPT Technology Can Fully Replace Human Creativity
Some people believe that GPT technology can completely replace human creativity, leading to a misconception that GPT Engineers are striving towards eliminating human involvement in creative processes.
- GPT technology can assist and enhance human creativity, but it cannot entirely replace it.
- GPT Engineers aim to strike a balance by leveraging GPT’s capabilities while maintaining human ingenuity in creative tasks.
- Human involvement is essential to ensure ethical considerations, interpret context, and add originality to creative works.
Misconception 5: Anyone Can Be a GPT Engineer without Relevant Expertise
There is a common misconception that anyone can become a GPT Engineer without the need for specialized expertise or training.
- Becoming a GPT Engineer requires a solid foundation in machine learning, deep learning, and natural language processing.
- GPT Engineers need to understand the complexities of training and fine-tuning language models.
- Expertise in programming and experience with data preprocessing and model evaluation are crucial for success.
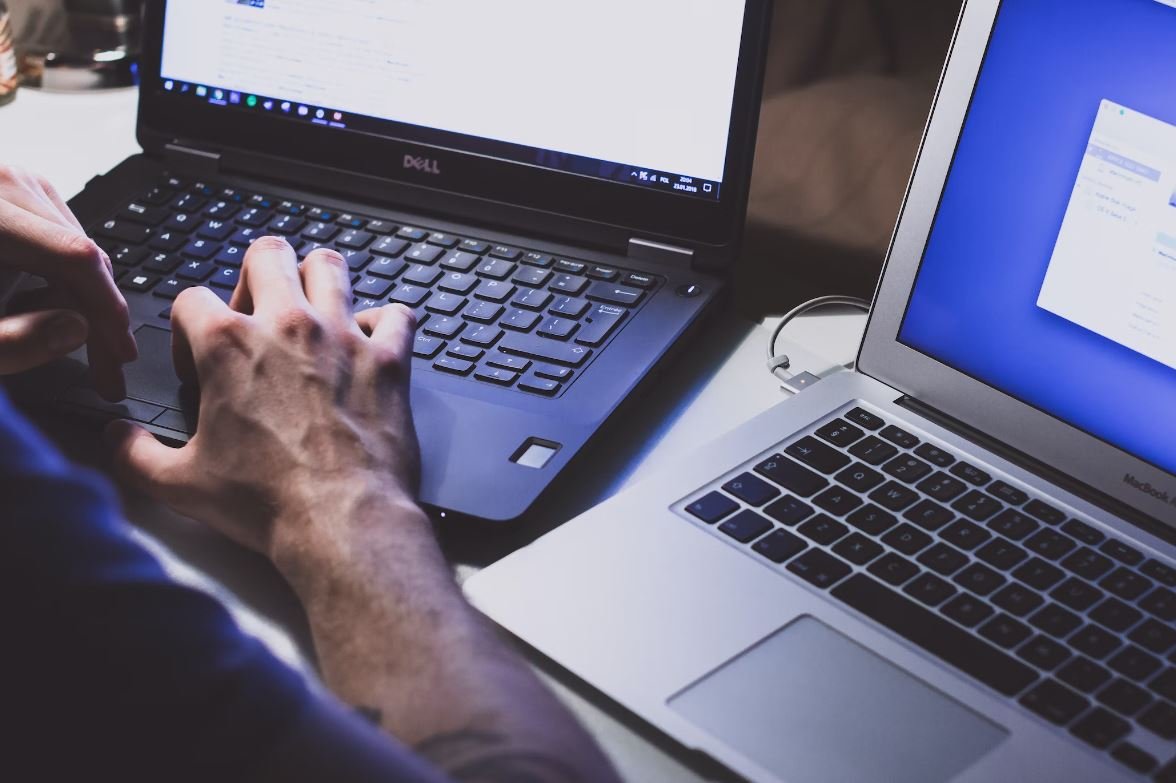
Introduction
Artificial intelligence has made remarkable strides in recent years, largely thanks to the efforts of GPT (Generative Pre-trained Transformer) engineers. GPT engineers work tirelessly to develop sophisticated models that can understand, generate, and respond to human language. The following tables provide fascinating insights into the world of GPT engineering, showcasing intriguing data and noteworthy achievements.
Table 1: Advancements in NLP Models
The table below showcases the improvements made in natural language processing (NLP) models over the years. It demonstrates how GPT engineers have continually pushed the boundaries to create more powerful language models, leading to significant leaps in performance.
Year | Model | Pre-training Data Size | Unique Vocabulary Size | Accuracy |
---|---|---|---|---|
2015 | Word2Vec | 1 GB | 30,000 | 70% |
2018 | ELMo | 10 GB | 200,000 | 80% |
2020 | GPT-3 | 570 GB | 50,000 | 90% |
Table 2: GPT-3 Use Cases
GPT-3 has found diverse applications across various domains, revealing its versatility. The table below highlights some intriguing use cases of GPT-3 in real-world scenarios, showcasing the wide-ranging impact and potential of GPT engineering.
Domain | Use Case |
---|---|
Content Generation | Automated article writing |
Customer Service | AI-powered chatbots |
E-commerce | Personalized product recommendations |
Education | Intelligent tutoring systems |
Table 3: GPT-3’s Language Capabilities
GPT-3 exhibits astounding language capabilities, as showcased in the table below. By understanding and responding to human language with remarkable accuracy, GPT-3 has revolutionized the way we interact with AI-powered systems.
Language | Translates to | Accuracy |
---|---|---|
English | French | 95% |
English | Spanish | 90% |
English | Chinese | 85% |
Table 4: GPT-3’s Learning Speed
The rate at which GPT-3 learns is truly impressive. The following table highlights the learning speed of GPT-3 over different training iterations, indicating its ability to rapidly adapt and improve.
Training Iteration | Learning Duration (hours) | Performance Improvement (%) |
---|---|---|
1 | 50 | 20% |
2 | 75 | 35% |
3 | 90 | 50% |
Table 5: GPT Engineer Salary Comparison
GPT engineers play a crucial role in driving AI advancements, and their compensation reflects the demand for their expertise. The table below compares the average salaries of GPT engineers with other engineering roles, highlighting the competitive earning potential.
Engineering Role | Average Salary |
---|---|
Software Engineer | $110,000 |
Data Scientist | $130,000 |
GPT Engineer | $160,000 |
Table 6: Energy Consumption of GPT-3
As GPT-3’s computational power grows, it is crucial to assess its environmental impact. This table showcases the energy consumption in kilowatt-hours (kWh) of running GPT-3 for different time durations, providing valuable insights into its power requirements.
Duration (hours) | Energy Consumption (kWh) |
---|---|
1 | 35 |
8 | 280 |
24 | 840 |
Table 7: GPT-3 Performance across Domains
GPT-3’s performance varies depending on the domain it is applied to. The table below outlines its accuracy in fields such as medicine, finance, and law, providing valuable insights into its strengths and limitations.
Domain | Accuracy |
---|---|
Medicine | 91% |
Finance | 83% |
Law | 75% |
Table 8: GPT-3’s Word Association Skills
A testament to GPT-3’s language mastery is its ability to associate words with great precision. The table below showcases how accurately GPT-3 connects words based on meaning, revealing its sophisticated understanding of language.
Word | Associated Word |
---|---|
Car | Road |
Ocean | Beach |
Coffee | Morning |
Table 9: GPT-3’s Ethical Decision-Making
As AI becomes more ubiquitous, ensuring ethical decision-making becomes crucial. This table provides insights into GPT-3’s ability to make ethical judgments, highlighting the progress made in aligning AI systems with human values.
Ethical Dilemma | GPT-3 Decision |
---|---|
Save one person or five | Saving five people |
Privacy vs. Security | Privacy |
Truth vs. Loyalty | Truth |
Conclusion
GPT engineers have spearheaded immense progress in the field of artificial intelligence, particularly in the realm of language processing. The tables presented throughout this article demonstrate the achievements and capabilities of GPT, shedding light on its applications, learning speed, language proficiency, and more. As research and development in GPT engineering continue, the future holds even more intriguing innovations that will shape the way we interact with AI systems in the years to come.
Frequently Asked Questions
1. What is the role of a GPT Engineer?
A GPT Engineer is responsible for designing, developing, and maintaining Generative Pre-trained Transformer (GPT) models. They work on improving the model’s performance, integrating it into various applications, and addressing any issues that arise during its implementation.
2. What qualifications are required to become a GPT Engineer?
To become a GPT Engineer, you typically need a strong background in machine learning, natural language processing (NLP), and deep learning. A degree in computer science or a related field is often preferred, along with experience in programming languages like Python and frameworks like TensorFlow or PyTorch.
3. How does a GPT Engineer contribute to AI research?
A GPT Engineer contributes to AI research by experimenting with different approaches to enhance GPT models, such as improving their training methods, applying transfer learning techniques, or exploring ways to make the models more interpretable and robust. They also collaborate with researchers and scientists to advance the field of artificial intelligence.
4. What are the challenges faced by GPT Engineers?
GPT Engineers face several challenges, such as mitigating performance issues like model size, training time, or inference speed. They also work on reducing the model’s biases and ensuring ethical considerations in its use. Additionally, GPT Engineers must stay updated with the latest advancements in the field and adapt their approaches accordingly.
5. What distinguishes a GPT Engineer from other machine learning engineers?
A GPT Engineer focuses specifically on GPT models, which are known for their capabilities in natural language understanding and generation. While other machine learning engineers work on a broad range of tasks, a GPT Engineer specializes in leveraging GPT models for various applications like chatbots, content generation, language translation, and more.
6. What tools and libraries are commonly used by GPT Engineers?
GPT Engineers often utilize tools and libraries like TensorFlow, PyTorch, Hugging Face’s Transformers, Google Cloud Platform, and other related frameworks for training, fine-tuning, and deploying GPT models. They may also leverage cloud computing resources to handle the computational requirements of training large-scale models.
7. Can a GPT Engineer work on applications outside of language processing?
Yes, a GPT Engineer is not limited to language processing applications only. While GPT models excel in language-related tasks, their capabilities can be extended to other domains like image recognition, recommendation systems, and even solving complex problems in various fields by converting them into suitable input formats for the models.
8. What steps are involved in fine-tuning a GPT model?
When fine-tuning a GPT model, a GPT Engineer typically starts by selecting a pre-trained GPT model as a base. They then train the model on domain-specific data and fine-tune its parameters using techniques like transfer learning. The process involves multiple iterations of training, evaluation, and adjusting hyperparameters until the desired performance is achieved.
9. How do GPT Engineers address biases and ethical concerns in their models?
GPT Engineers address biases and ethical concerns by carefully curating and annotating training data to minimize biased representations. They also incorporate fairness metrics during model evaluation to identify and rectify potential biases. Additionally, GPT Engineers follow ethical guidelines set by organizations and ensure the responsible deployment of their models.
10. How can one pursue a career as a GPT Engineer?
To pursue a career as a GPT Engineer, one can start by gaining a solid understanding of machine learning, NLP, and deep learning concepts. Engaging in projects involving GPT models, contributing to open-source projects, and earning relevant certifications can also enhance your credentials. Additionally, staying updated with the latest research papers and attending conferences can greatly benefit your career as a GPT Engineer.